In the rapidly evolving landscape of artificial intelligence and machine learning, the demand for skilled professionals who can navigate the complexities of cloud-based ML solutions is surging. The AWS Certified Machine Learning Engineer – Associate certification is a testament to your proficiency in designing, implementing, deploying, and maintaining machine learning workloads on the world’s leading cloud platform. This rigorous exam validates your ability to leverage the vast array of AWS services to build scalable, secure, and cost-effective ML applications. This AWS Certified Machine Learning Engineer – Associate Cheat Sheet is designed to be your essential companion as you prepare for the AWS Certified Machine Learning Engineer – Associate exam.
We’ll delve into the core machine learning concepts, explore the intricate workings of key AWS services like Amazon SageMaker, and address the critical aspects of security, cost optimization, and deployment. This guide covers the exam’s breadth, from fundamental algorithms to advanced deployment strategies.
Exam Importance & Benefits
Machine learning (ML) is transforming industries, and AWS offers a robust ecosystem for cloud-based ML solutions. The AWS Certified Machine Learning Engineer – Associate certification validates expertise in deploying, managing, and optimizing ML workflows on AWS, enhancing career growth, industry recognition, and professional credibility.
- Validating Specialized Expertise
- Proficiency in AWS ML Services: Demonstrates the ability to use Amazon SageMaker, AI APIs, and data analytics tools for scalable ML solutions.
- End-to-End ML Lifecycle Mastery: Confirms expertise in data preprocessing, model training, deployment, and monitoring.
- Business-Oriented ML Solutions: Showcases the ability to align ML implementations with business goals.
- Career Advancement and Increased Earning Potential
- Better Job Prospects: Boosts employability in high-demand roles like ML Engineer and AI Solutions Architect.
- Higher Salaries: Certified professionals are often compensated more due to proven expertise.
- Promotion Opportunities: Opens doors to leadership and specialized roles.
- Industry Recognition: Adds credibility and enhances professional reputation.
- Building Credibility and Professional Reputation
- Authority in AWS ML: Validates skills and establishes expertise.
- Professional Networking: Connects you with AWS experts and certified professionals.
- Confidence Boost: Strengthens self-assurance in handling ML projects.
- Staying Current with Industry Best Practices
- Ongoing Learning: Keeps you updated with the latest AWS ML advancements.
- Technological Adaptability: Ensures proficiency with evolving AWS services.
- Standardized Methodologies: Promotes adherence to industry best practices.
- Contribution to Organizational Success
- Efficiency and Innovation: Enhances ML model development and optimization.
- Cost Reduction: Optimizes AWS resource utilization, reducing infrastructure costs.
- Data-Driven Decision-Making: Empowers businesses with robust ML solutions.
- Risk Mitigation: Ensures security and compliance in ML deployments.
- Access to AWS Certification Benefits
- Global AWS Certified Community: Provides collaboration and networking opportunities.
- Exclusive Discounts & Offers: Includes savings on training and events.
- Digital Badges: Enhances online professional visibility.
Scope of Machine Learning Engineer – Associate Cheat Sheet
The AWS Certified Machine Learning Engineer – Associate Exam requires a strong understanding of machine learning (ML) principles, AWS ML services, and best practices for deploying scalable solutions. This cheat sheet serves as a concise yet comprehensive study guide, designed to streamline your preparation by summarizing key concepts, AWS services, and exam-focused strategies. While it provides structured insights, it is not a substitute for hands-on experience or official AWS documentation.
– What This Cheat Sheet Covers
- Machine Learning Fundamentals: A breakdown of essential ML concepts, including supervised, unsupervised, and reinforcement learning, deep learning principles, and key evaluation metrics.
- AWS Machine Learning Services: Detailed coverage of Amazon SageMaker, AI-powered AWS services, and the supporting data and compute infrastructure crucial for ML workflows.
- Security, Cost Optimization, and Deployment Strategies: Best practices for securing ML models, optimizing resource usage, and deploying solutions efficiently on AWS.
- Exam-Focused Terminology and Key Concepts: Highlights of frequently tested terms and concepts to align your understanding with exam expectations.
- Structured Content for Effective Learning: A well-organized format that facilitates quick review and knowledge retention.
– How to Best Use This Cheat Sheet
- Use it as a Study Guide: Regularly review the content to reinforce critical concepts.
- Enhance Learning with Hands-on Practice: Explore AWS services through the AWS Management Console and practice implementing ML solutions.
- Supplement with Official AWS Documentation: Cross-reference AWS documentation to deepen your understanding of complex topics.
- Take Practice Exams: Identify knowledge gaps and improve retention by attempting mock exams.
- Understand Service Relationships: Focus on how different AWS services integrate to build end-to-end ML pipelines, a key aspect of the exam.
AWS Certified Machine Learning Engineer – Associate Cheat Sheet
The AWS Certified Machine Learning Engineer – Associate Cheat Sheet 2025 is a concise yet comprehensive study aid designed to streamline your exam preparation. It covers key machine learning concepts, AWS ML services, security best practices, cost optimization, and deployment strategies. While not a substitute for hands-on practice or official documentation, this cheat sheet helps reinforce essential topics, making it easier to review and retain critical information.
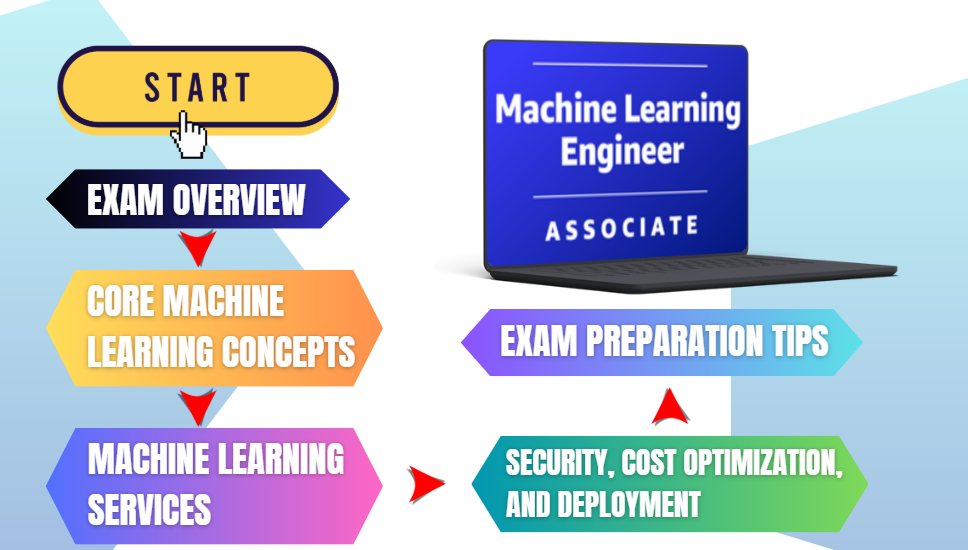
Exam Overview
The AWS Certified Machine Learning Engineer – Associate certification validates a candidate’s ability to design, deploy, and manage machine learning (ML) solutions on AWS. It demonstrates technical proficiency in implementing ML workloads in production, operationalizing models, and optimizing ML pipelines. This certification enhances professional credibility and career prospects, positioning candidates for in-demand roles in machine learning and AI.
– Exam Scope and Key Skills Assessed
The MLA-C01 exam evaluates a candidate’s expertise in:
- Data Preparation: Ingesting, transforming, validating, and preparing data for ML models.
- Model Development: Selecting modeling approaches, training models, tuning hyperparameters, analyzing model performance, and managing model versions.
- Deployment & Scaling: Choosing deployment infrastructure, provisioning compute resources, and configuring auto-scaling.
- CI/CD Automation: Setting up continuous integration and continuous delivery (CI/CD) pipelines to streamline ML workflows.
- Monitoring & Security: Tracking models, data, and infrastructure performance, ensuring security through access controls and compliance best practices.
– Target Audience
This certification is ideal for professionals with at least one year of experience using Amazon SageMaker and other AWS services for ML engineering. Suitable candidates typically have backgrounds in roles such as:
- Backend Software Developers
- DevOps Engineers
- Data Engineers
- Data Scientists
– Recommended Knowledge and Skills
General IT Knowledge:
- Fundamental understanding of ML algorithms and their applications
- Data engineering principles, including data formats, ingestion, and transformation
- Querying and transforming data for ML pipelines
- Software engineering best practices for modular, reusable code
- Experience with CI/CD pipelines and infrastructure as code (IaC)
- Familiarity with cloud and on-premises ML resource provisioning and monitoring
- Version control and code repository management for CI/CD workflows
AWS-Specific Knowledge:
- Expertise in Amazon SageMaker for model development and deployment
- Understanding of AWS data storage and processing services for ML
- Experience deploying ML applications and infrastructure on AWS
- Proficiency in AWS monitoring tools for logging and troubleshooting
- Knowledge of AWS automation services for CI/CD pipeline orchestration
- Strong grasp of AWS security best practices, including identity and access management (IAM), encryption, and data protection
– Exam Format
- The AWS Certified Machine Learning Engineer – Associate certification validates an individual’s expertise in designing, implementing, and managing machine learning (ML) workloads on AWS.
- This associate-level exam is designed for professionals with at least one year of experience using Amazon SageMaker and other AWS ML services, including backend software developers, DevOps engineers, data engineers, MLOps engineers, and data scientists.
- The exam consists of 65 questions and lasts 130 minutes, with a minimum passing score of 720.
- Candidates will encounter various question types, including multiple choice, multiple response, ordering, matching, and case studies, each assessing their ability to build, deploy, and maintain ML solutions.
- Available in English, Japanese, Korean, and Simplified Chinese, the exam can be taken at a Pearson VUE testing center or through an online proctored format.
- Achieving this certification demonstrates proficiency in ML model training, deployment, automation, security, and performance optimization on AWS, making it a valuable credential for those looking to advance their careers in machine learning and cloud-based AI solutions.
Core Machine Learning Concepts
Machine Learning (ML) is the driving force behind modern artificial intelligence (AI) applications, enabling systems to automatically learn from data and improve their performance without explicit programming. As organizations increasingly adopt cloud-based ML solutions, a strong foundation in key ML principles is essential for professionals working with AWS services.
This section provides a structured overview of fundamental ML concepts, including various learning paradigms, data preprocessing techniques, model evaluation strategies, and deployment best practices. These foundational topics are critical for professionals preparing for the AWS Certified Machine Learning Engineer – Associate exam, as they form the basis for designing, implementing, and maintaining ML solutions in production environments.
– Machine Learning Fundamentals
Machine learning techniques can be broadly classified into three major categories: supervised learning, unsupervised learning, and reinforcement learning. Each category has distinct use cases, methodologies, and evaluation metrics. Additionally, deep learning, a specialized subset of ML, leverages artificial neural networks to handle complex data patterns and automate decision-making processes.
1. Supervised Learning
Supervised learning involves training ML models using labeled datasets, where each data point has a known output. This approach is widely used in predictive modeling tasks, such as sales forecasting, spam detection, and medical diagnosis.
- Regression: This technique is used to predict continuous numerical values based on historical data. For instance, it can estimate housing prices based on features like square footage, location, and amenities. Common regression algorithms include Linear Regression, Polynomial Regression, and Support Vector Regression (SVR). Model performance is typically evaluated using metrics such as Mean Squared Error (MSE), Root Mean Squared Error (RMSE), Mean Absolute Error (MAE), and R-squared (R²).
- Classification: In contrast to regression, classification is used to predict categorical labels, such as detecting fraudulent transactions or identifying spam emails. Popular classification algorithms include Logistic Regression, Decision Trees, Random Forests, Support Vector Machines (SVM), Naive Bayes, k-Nearest Neighbors (k-NN), and Gradient Boosting methods like XGBoost and LightGBM. The performance of classification models is assessed using metrics such as Accuracy, Precision, Recall, F1-score, AUC-ROC (Area Under the Receiver Operating Characteristic curve), and Confusion Matrix.
2. Unsupervised Learning
Unsupervised learning deals with unlabeled data, where the goal is to identify hidden patterns or structures within the dataset. This approach is useful for customer segmentation, anomaly detection, and topic modeling.
- Clustering: Clustering algorithms group similar data points together based on shared characteristics. For example, businesses use clustering techniques to segment customers based on purchasing behavior. Common clustering algorithms include k-Means, Hierarchical Clustering, DBSCAN (Density-Based Spatial Clustering of Applications with Noise), and Gaussian Mixture Models (GMM). Evaluation metrics such as Silhouette Score, Davies-Bouldin Index, and Calinski-Harabasz Index help assess clustering performance.
- Dimensionality Reduction: High-dimensional data can be challenging to analyze and visualize. Dimensionality reduction techniques aim to preserve meaningful patterns while reducing the number of input features. Popular techniques include Principal Component Analysis (PCA), t-SNE (t-distributed Stochastic Neighbor Embedding), and Singular Value Decomposition (SVD). These methods are particularly useful in areas like image compression and feature selection for ML models.
3. Reinforcement Learning
Reinforcement learning (RL) is an advanced ML approach where an agent learns to take optimal actions in an environment by maximizing cumulative rewards. This method is widely used in robotics, autonomous vehicles, gaming, and algorithmic trading.
Key RL components include Agents (decision-makers), Actions (available choices), Rewards (feedback signals), and Policies (rules governing actions). Popular RL techniques include Q-learning, Deep Q-Networks (DQN), and Policy Gradients. AWS services like AWS DeepRacer provide practical applications of RL in autonomous vehicle simulations.
4. Deep Learning
Deep learning, a subset of ML, utilizes artificial neural networks (ANNs) with multiple layers to process and analyze complex datasets. It is particularly effective for image recognition, speech processing, and natural language understanding.
- Artificial Neural Networks (ANNs): Comprising interconnected neurons, ANNs are widely used in various applications such as fraud detection and medical diagnosis. Key components include activation functions (ReLU, Sigmoid, Tanh), loss functions (MSE, Cross-Entropy), and optimization algorithms (SGD, Adam, RMSprop).
- Convolutional Neural Networks (CNNs): Primarily used for image and video processing, CNNs employ convolutional layers to extract spatial features, pooling layers to reduce dimensionality, and fully connected layers for classification.
- Recurrent Neural Networks (RNNs): Designed for sequential data, RNNs are widely used in time series forecasting, speech recognition, and machine translation. Variants like Long Short-Term Memory (LSTM) networks and Gated Recurrent Units (GRU) help mitigate issues like vanishing gradients.
- Transformers: The latest advancement in deep learning, transformers power state-of-the-art natural language processing (NLP) models like BERT (Bidirectional Encoder Representations from Transformers) and GPT (Generative Pre-trained Transformer). They leverage attention mechanisms to process long sequences efficiently, revolutionizing fields like chatbots, text summarization, and code generation.
– Data Preprocessing
Data preprocessing is a crucial step in ML workflows, ensuring that raw data is cleaned, transformed, and structured for optimal model performance. High-quality data enhances model accuracy, reduces biases, and accelerates training times.
- Data Cleaning: Involves handling missing values through techniques like mean/mode imputation or deletion, removing duplicate records, and detecting outliers using statistical methods such as z-score analysis and IQR filtering.
- Feature Engineering: Improves model accuracy by transforming raw data into meaningful representations. It includes feature scaling (standardization, normalization), feature encoding (One-Hot Encoding, Label Encoding), and dimensionality reduction techniques (PCA, t-SNE).
- Data Transformation: Transformations such as log transformations, Box-Cox transformations, and power transformations modify data distributions to improve model stability.
– Model Evaluation and Validation
Evaluating ML models ensures that they generalize well to new data and do not suffer from overfitting or underfitting.
- Cross-Validation
- Cross-validation techniques, including k-fold, stratified k-fold, and leave-one-out cross-validation, help assess model generalization.
- Hyperparameter Tuning
- Hyperparameters control model learning behavior and require optimization using techniques like grid search, random search, and Bayesian optimization.
- Bias-Variance Trade-off
- Striking a balance between bias (underfitting) and variance (overfitting) is essential for achieving robust models.
- Overfitting and Underfitting
- Regularization techniques like L1 (Lasso) and L2 (Ridge), along with early stopping, help prevent models from memorizing noise instead of learning patterns.
– Model Deployment and Monitoring
Deploying ML models into production requires continuous monitoring and maintenance to ensure performance and reliability.
- A/B Testing
- A/B testing compares different model versions to determine the most effective one based on real-world performance metrics.
- Model Drift Detection
- Over time, models can experience data drift (changes in input data distribution) or concept drift (shifts in the relationship between input and output variables), requiring proactive detection and retraining.
- Explainability and Interpretability
- Understanding model decisions is crucial for trust and transparency. Techniques like SHAP values, LIME, and feature importance analysis provide insights into model predictions.
AWS Machine Learning Services
AWS offers a comprehensive suite of machine learning (ML) services designed to simplify model development, deployment, and management. These services cater to various needs, from model training and inference to automated machine learning and AI-driven applications. AWS Machine Learning services are divided into key categories: Amazon SageMaker, AWS AI Services, AWS Data Services, and AWS Compute Services. Understanding these services is crucial for professionals aiming to build scalable ML solutions on AWS.
– Amazon SageMaker
Amazon SageMaker is a fully managed service that streamlines the entire machine learning lifecycle, providing an integrated environment for data preparation, model training, hyperparameter tuning, deployment, and monitoring. With a range of built-in tools, SageMaker simplifies ML workflows and enhances productivity.
1. SageMaker Studio
SageMaker Studio is an integrated development environment (IDE) for ML, offering a unified interface for managing all ML workflows. It includes features such as:
- Jupyter Notebooks for interactive coding.
- Experiment tracking, debugging, and profiling tools for better model analysis.
- SageMaker Canvas, a no-code ML solution, enabling business analysts to build models without programming expertise.
2. SageMaker Notebook Instances
SageMaker provides fully managed Jupyter notebooks, allowing users to experiment with different ML models efficiently. These notebooks support:
- Various instance types, including CPU and GPU configurations for scalable computing power.
- Lifecycle configurations, enabling customization of notebook environments based on project needs.
3. SageMaker Training Jobs
Model training in SageMaker supports both built-in and custom algorithms, offering flexibility in handling diverse ML problems. Features include:
- Built-in algorithms like Linear Learner and XGBoost.
- Custom algorithms using the “Bring Your Own Container (BYOC)” approach.
- Distributed training strategies, such as data parallelism, model parallelism, and pipeline parallelism.
- SageMaker Debugger for real-time insights into model training.
- Hyperparameter tuning (SageMaker Autotuning) for optimizing model performance.
- Managed Spot Training to reduce costs by using spare AWS capacity.
4. SageMaker Inference
SageMaker offers multiple inference options for deploying models:
- Real-time inference (SageMaker Endpoints): Enables instant predictions with auto-scaling capabilities and multi-model endpoints.
- Batch Transform: Used for large-scale, offline batch predictions.
- Serverless Inference: Automatically scales down to zero when not in use, reducing costs for infrequent predictions.
- Inference Recommender: Assists in selecting the optimal instance type for inference workloads.
5. SageMaker Model Monitor
Ensuring model performance over time is critical. SageMaker Model Monitor detects:
- Model drift, including data and concept drift.
- Performance degradation, issuing alerts when models deviate from expected accuracy.
6. SageMaker Clarify
SageMaker Clarify addresses bias detection and explainability, ensuring fairness in ML models by:
- Identifying potential bias in training datasets.
- Explaining predictions using SHAP values and feature importance.
7. SageMaker Pipelines
To streamline ML workflows, SageMaker Pipelines automates:
- Training, evaluation, and deployment.
- CI/CD integration, ensuring continuous improvement of ML models.
8. SageMaker Feature Store
Feature engineering is a crucial step in ML, and SageMaker Feature Store provides:
- A centralized repository for storing and managing features.
- Online and offline feature stores for real-time and batch predictions.
9. SageMaker Ground Truth
SageMaker Ground Truth simplifies data labeling with:
- Human and automated labeling workflows.
- Support for large-scale labeling jobs across various data types.
10. SageMaker Autopilot
For those looking for an AutoML solution, SageMaker Autopilot offers:
- Automated model selection and hyperparameter tuning.
- Performance comparisons across multiple algorithms.
11. SageMaker Neo
SageMaker Neo enables model optimization for edge devices, ensuring:
- Efficient deployment on hardware-constrained environments.
- Optimized performance across multiple device types.
12. SageMaker JumpStart
SageMaker JumpStart accelerates ML adoption with:
- Pre-trained models for common use cases.
- Pre-built ML solutions to quickly deploy AI-powered applications.
– AWS AI Services
AWS offers a wide range of AI services designed to help businesses integrate advanced machine learning capabilities without requiring deep expertise in AI. These services provide ready-to-use models for image recognition, natural language processing, speech synthesis, and more.
- Amazon Rekognition
- A powerful image and video analysis service that provides features like object detection, facial recognition, text extraction, and content moderation.
- Use cases: Security authentication, content moderation for social media, automated metadata tagging, and customer sentiment analysis from images.
- Amazon Comprehend
- A natural language processing (NLP) service that extracts key phrases, sentiments, language, and entity recognition from text data.
- Use cases: Analyzing customer feedback, topic modeling for documents, automated ticket classification in customer support, and legal or healthcare document processing.
- Amazon Translate
- A neural machine translation service that enables real-time, high-quality language translation across multiple languages.
- Use cases: Website localization, multilingual chatbot responses, cross-border eCommerce, and document translation.
- Amazon Transcribe
- A speech-to-text service that automatically converts spoken language into written text with support for multiple languages.
- Use cases: Meeting transcription, automated call center analytics, closed captioning for videos, and voice search applications.
- Amazon Polly
- A text-to-speech (TTS) service that converts written content into lifelike speech using deep learning technology.
- Features include multiple voice styles, customizable speech synthesis, and speech-enhanced applications.
- Use cases: Audiobook narration, automated customer service interactions, voice-enabled applications, and accessibility tools for visually impaired users.
- Amazon Lex
- A conversational AI service used to build chatbots and virtual assistants with automatic speech recognition (ASR) and natural language understanding (NLU).
- Use cases: Customer service automation, virtual assistants, interactive voice response (IVR) systems, and enterprise chatbots.
- Amazon Personalize
- A machine learning-based recommendation engine that provides personalized content suggestions.
- Use cases: E-commerce product recommendations, personalized movie and music recommendations, and customized news feeds.
- Amazon Forecast
- A time-series forecasting service that leverages ML to generate accurate demand predictions based on historical data.
- Use cases: Sales forecasting, inventory optimization, financial planning, and resource allocation.
- Amazon Kendra
- An enterprise search service that enables organizations to search and retrieve information from unstructured text data.
- Use cases: Knowledge management systems, legal and compliance document searches, and customer support knowledge bases.
– AWS Data Services
Managing and preparing data is a critical step in the machine learning workflow. AWS offers various data services to store, process, and transform data efficiently for ML applications.
- Amazon S3 (Simple Storage Service)
- A highly scalable object storage service for storing raw datasets, training data, and ML model artifacts.
- Features include lifecycle policies, security with IAM roles, versioning, and intelligent-tiering for cost efficiency.
- Amazon RDS (Relational Database Service)
- A managed relational database service that supports multiple database engines, including MySQL, PostgreSQL, and SQL Server.
- Used for structured data storage, transaction processing, and analytical workloads in ML pipelines.
- Amazon DynamoDB
- A fully managed NoSQL database that offers high-speed, low-latency access to ML-related data.
- Ideal for real-time recommendation engines, session storage, and fraud detection applications.
- AWS Glue
- A serverless data integration service used for ETL (Extract, Transform, Load) processes.
- Allows automated schema discovery, data cataloging, and transformation to prepare data for ML training.
- Amazon Athena
- A serverless query engine that enables users to run SQL queries directly on structured and unstructured data stored in Amazon S3.
- Commonly used for data exploration, preprocessing, and quick analysis before model training.
- Amazon EMR (Elastic MapReduce)
- A managed Hadoop and Spark service that processes large-scale datasets for ML applications.
- Use cases: Big data processing, large-scale data transformations, and distributed ML model training.
- AWS Lake Formation
- A service that simplifies building, securing, and managing data lakes.
- Allows organizations to aggregate structured and unstructured data from different sources and make it ML-ready.
– AWS Compute Services
Compute services play a crucial role in training, deploying, and running ML models efficiently. AWS provides multiple compute solutions optimized for different workloads, from serverless functions to high-performance GPU instances.
- Amazon EC2 (Elastic Compute Cloud)
- Provides scalable virtual machines (instances) for ML training and inference.
- Includes specialized GPU instances such as:
- P3 and P4 Instances – Optimized for high-performance ML training with NVIDIA GPUs.
- G4 and G5 Instances – Designed for inference workloads and low-latency model serving.
- Inf Instances – Optimized for deep learning inference using AWS Inferentia chips.
- AWS Lambda
- A serverless compute service that automatically runs code in response to events.
- Commonly used for preprocessing ML data, handling inference requests, and triggering ML workflows.
- Eliminates the need for server management, making it a cost-efficient choice for lightweight ML tasks.
- Amazon ECS (Elastic Container Service) and Amazon EKS (Elastic Kubernetes Service)
- ECS: A container orchestration service that enables users to run ML workloads in Docker containers.
- EKS: A managed Kubernetes service for deploying, managing, and scaling ML applications in a Kubernetes environment.
- Use cases: Running TensorFlow and PyTorch models in containers, managing batch inference jobs, and deploying ML microservices.
- AWS Batch
- A fully managed service that runs batch computing jobs, including large-scale ML model training and data preprocessing.
- Supports parallel processing and automatic scaling of compute resources based on workload demand.
- AWS Elastic Inference
- Allows users to attach GPU acceleration to Amazon EC2 and SageMaker instances for cost-effective deep learning inference.
- Reduces costs compared to using full GPU instances while maintaining inference speed.
- AWS Inferentia
- A custom ML inference chip designed to optimize deep learning inference workloads at a lower cost than traditional GPUs.
- Used with Amazon EC2 Inf1 instances for large-scale AI model deployment.
Security, Cost Optimization, and Deployment
As organizations increasingly adopt AWS for machine learning (ML) workloads, ensuring security, optimizing costs, and implementing efficient deployment strategies are critical for success. AWS provides a comprehensive suite of security controls, cost-saving mechanisms, and deployment options to help businesses streamline their ML workflows. This section explores best practices for securing ML environments, managing costs effectively, and deploying models with reliability and scalability.
– Security Best Practices
Security is a top priority when deploying ML models on AWS. AWS provides multiple layers of security to protect data, models, and infrastructure. Implementing best practices in identity and access management, encryption, and network security ensures robust protection against threats.
1. IAM Roles and Policies
AWS Identity and Access Management (IAM) enables fine-grained access control for ML services like SageMaker, EC2, and S3.
- The Principle of Least Privilege should be followed, granting users and services only the permissions they need.
- Custom IAM roles should be created for different ML services to segregate access and minimize security risks.
- Service Control Policies (SCPs) can enforce security constraints at an organizational level to prevent unauthorized changes.
2. Encryption (At Rest and In Transit)
Data encryption is essential for protecting sensitive ML datasets and models.
- S3 encryption options include SSE-S3, SSE-KMS, and SSE-C, ensuring data remains secure in storage.
- RDS and SageMaker Notebook Encryption protects stored datasets and training results.
- HTTPS encryption for SageMaker endpoints ensures secure API communication.
- Transport Layer Security (TLS) encrypts data in transit to prevent interception and tampering.
3. Virtual Private Cloud (VPC) and Security Groups
Securing network access helps prevent unauthorized access to ML resources.
- Creating secure VPCs for ML workloads isolates traffic and enhances security.
- Security groups define inbound and outbound traffic rules, limiting access to essential services.
- Private subnets can be used for resources handling sensitive data, reducing exposure to the public internet.
- VPC endpoints enable private communication with AWS services, eliminating the need for internet access.
4. AWS Key Management Service (KMS)
AWS KMS provides centralized key management for data encryption.
- Encryption keys can be used to secure ML models, training data, and stored results.
- S3, RDS, and SageMaker integration with KMS ensures all sensitive data remains protected.
5. Data Encryption in S3 and RDS
To maintain data confidentiality, organizations should implement structured encryption strategies.
- Using automated key rotation for long-term security.
- Auditing data access to detect unauthorized attempts.
- Enforcing strict access control mechanisms for sensitive datasets.
– Cost Optimization
Managing ML workloads efficiently on AWS can significantly reduce costs. Selecting the right instance types, leveraging spot instances, and optimizing storage are key strategies.
1. Choosing the Right Instance Types
Different ML workloads require different compute resources:
- GPU instances (e.g., P3, P4) are best suited for deep learning training but should be used only when necessary.
- Inferentia-based Inf instances provide cost-effective inference for deep learning models.
- SageMaker instance selection should align with workload requirements to balance performance and cost.
2. Leveraging Spot Instances for Training
AWS Spot Instances offer significant savings by using spare EC2 capacity.
- Ideal for training ML models where temporary interruptions can be managed.
- Implementing checkpointing mechanisms allows resumption from the last saved state.
3. SageMaker Managed Spot Training
SageMaker supports automatic use of spot instances, reducing training costs without requiring manual intervention.
4. Auto Scaling for Endpoints
- Dynamically scaling SageMaker endpoints based on incoming traffic optimizes resource usage.
- Reducing instance count during low-demand periods minimizes expenses.
5. Storage Optimization with S3 Lifecycle Policies
- Moving infrequently accessed data to lower-cost storage classes (e.g., S3 Glacier, Intelligent-Tiering).
- Automatically deleting outdated models and datasets using lifecycle rules.
6. Cost Optimization for Serverless Inference
- Understanding the cost structure of serverless ML inference helps in cost planning.
- Minimizing cold starts in serverless environments ensures cost-effective deployments.
– Deployment Strategies
Ensuring seamless and scalable deployment is crucial for maintaining ML model performance and reliability. AWS provides multiple deployment techniques to minimize downtime and enhance model versioning.
1. Blue/Green Deployments
A blue/green deployment strategy minimizes downtime and risk during model updates.
- The new model version (green) is deployed alongside the current version (blue).
- Traffic is gradually switched to the new version after validation.
- If issues arise, traffic is easily reverted to the stable version.
2. Canary Deployments
This approach involves incremental model rollout:
- Deploying to a small subset of traffic before full deployment.
- Monitoring model performance and rolling back if issues are detected.
3. CI/CD Pipelines with AWS CodePipeline and CodeBuild
Automating ML model deployment using CI/CD pipelines enhances efficiency.
- AWS CodePipeline and CodeBuild streamline the build, test, and deployment phases.
- Integration with SageMaker enables continuous model updates.
- AWS CodeDeploy helps manage endpoint upgrades.
4. Model Deployment using Docker Containers
Containerizing models ensures consistency across different environments.
- Amazon Elastic Container Registry (ECR) stores model images.
- Amazon ECS and EKS manage scalable deployments using Docker.
5. SageMaker Pipelines for Model Deployment
AWS SageMaker Pipelines automate the end-to-end ML workflow.
- Ensures repeatable, auditable, and scalable deployment processes.
- Reduces manual intervention in deployment, improving operational efficiency.
6. Shadow Testing
Shadow testing allows evaluation of new models without affecting live users.
- Sending production traffic to the new model in parallel.
- Analyzing results before fully switching over.
- Ensuring reliability before replacing the existing model.
AWS Machine Learning Engineer – Associate Exam Preparation Tips
Preparing for the AWS Certified Machine Learning Engineer – Associate exam requires a strategic approach that combines hands-on practice, theoretical understanding, and familiarity with AWS services. This section provides detailed insights into how candidates can enhance their preparation and improve their chances of success.
– Hands-on Experience
A practical understanding of AWS Machine Learning services is crucial for the exam. Hands-on experience will not only solidify theoretical concepts but also help candidates apply them in real-world scenarios.
- Practical Projects: Work on end-to-end machine learning projects using AWS SageMaker. This should involve all stages of ML workflows, from data ingestion and preprocessing to model training, deployment, and monitoring. Experiment with different ML algorithms, instance types, and optimization techniques to understand performance trade-offs.
- AWS Console Exploration: Spend time navigating the AWS Management Console, particularly SageMaker, AI services, and supporting data services. Understanding how to configure and manage different AWS settings will be beneficial when tackling scenario-based questions in the exam.
- Real-World Scenarios: Participate in Kaggle competitions, open-source ML projects, or work on real-world case studies that incorporate AWS machine learning services. These activities will enhance problem-solving skills and prepare candidates for the type of challenges they might face in the exam.
– AWS Documentation and Whitepapers
AWS provides comprehensive documentation and whitepapers that cover machine learning best practices, architectural guidelines, and service-specific details.
- Official AWS Documentation: Reviewing the official AWS documentation for SageMaker and other AI services is essential. Focus on key features, limitations, best practices, and frequently asked questions to gain in-depth knowledge.
- AWS Whitepapers: AWS offers a range of whitepapers on topics such as machine learning on AWS, security best practices, and cost optimization. Candidates should particularly focus on papers that align with the exam’s domains.
- AWS Well-Architected Framework: Understanding the AWS Well-Architected Framework, especially its five pillars—cost optimization, security, operational excellence, reliability, and performance efficiency—is crucial for designing scalable and efficient ML solutions.
– Focus on Exam Objectives
The AWS Certified Machine Learning Engineer – Associate exam assesses a candidate’s ability to design, implement, and optimize machine learning (ML) solutions using AWS services. Understanding the core objectives of the exam is crucial for effective preparation. The exam is structured around the following domains:
Domain 1: Data Preparation for Machine Learning (ML)
Task Statement 1.1: Ingest and store data.
Knowledge of:
- Data formats and ingestion mechanisms (for example, validated and non-validated formats, Apache Parquet, JSON, CSV, Apache ORC, Apache Avro, RecordIO) (AWS Documentation: Data ingestion methods, Common Data Formats for Training)
- How to use the core AWS data sources (for example, Amazon S3, Amazon Elastic File System [Amazon EFS], Amazon FSx for NetApp ONTAP) (AWS Documentation: What is Amazon FSx for NetApp ONTAP?, Getting started with Amazon FSx for NetApp ONTAP)
- How to use AWS streaming data sources to ingest data (for example, Amazon Kinesis, Apache Flink, Apache Kafka) (AWS Documentation: Apache Flink, Add streaming data sources to Managed Service for Apache Flink)
- AWS storage options, including use cases and tradeoffs (AWS Documentation: Storage, Choosing an AWS storage service)
Skills in:
- Extracting data from storage (for example, Amazon S3, Amazon Elastic Block Store [Amazon EBS], Amazon EFS, Amazon RDS, Amazon DynamoDB) by using relevant AWS service options (for example, Amazon S3 Transfer Acceleration, Amazon EBS Provisioned IOPS) (AWS Documentation: S3 Transfer Acceleration, Exporting DB snapshot data to Amazon S3 for Amazon RDS)
- Choosing appropriate data formats (for example, Parquet, JSON, CSV, ORC) based on data access patterns (AWS Documentation: Choose data formatting based on your data access pattern)
- Ingesting data into Amazon SageMaker Data Wrangler and SageMaker Feature Store (AWS Documentation: Data sources and ingestion)
- Merging data from multiple sources (for example, by using programming techniques, AWS Glue, Apache Spark)
- Troubleshooting and debugging data ingestion and storage issues that involve capacity and scalability
- Making initial storage decisions based on cost, performance, and data structure
Task Statement 1.2: Transform data and perform feature engineering.
Knowledge of:
- Data cleaning and transformation techniques (for example, detecting and treating outliers, imputing missing data, combining, deduplication) (AWS Documentation: Transform Data)
- Feature engineering techniques (for example, data scaling and standardization, feature splitting, binning, log transformation, normalization) (AWS Documentation: Feature engineering)
- Encoding techniques (for example, one-hot encoding, binary encoding, label encoding, tokenization) (AWS Documentation: Transform Data)
- Tools to explore, visualize, or transform data and features (for example, SageMaker Data Wrangler, AWS Glue, AWS Glue DataBrew) (AWS Documentation: What is AWS Glue DataBrew?)
- Services that transform streaming data (for example, AWS Lambda, Spark) (AWS Documentation: Working with streaming data on AWS)
- Data annotation and labeling services that create high-quality labeled datasets (AWS Documentation: Use Amazon SageMaker Ground Truth Plus to Label Data)
Skills in:
- Transforming data by using AWS tools (for example, AWS Glue, AWS Glue DataBrew, Spark running on Amazon EMR, SageMaker Data Wrangler)
- Creating and managing features by using AWS tools (for example, SageMaker Feature Store)
- Validating and labeling data by using AWS services (for example, SageMaker Ground Truth, Amazon Mechanical Turk)
Task Statement 1.3: Ensure data integrity and prepare data for modeling.
Knowledge of:
- Pre-training bias metrics for numeric, text, and image data (for example, class imbalance [CI], difference in proportions of labels [DPL]) (AWS Documentation: Pre-training Bias Metrics, Difference in Proportions of Labels (DPL))
- Strategies to address CI in numeric, text, and image datasets (for example, synthetic data generation, resampling) (AWS Documentation: Prepare data for model building)
- Techniques to encrypt data (AWS Documentation: Encrypting Data-at-Rest and Data-in-Transit)
- Data classification, anonymization, and masking
- Implications of compliance requirements (for example, personally identifiable information [PII], protected health information [PHI], data residency) (AWS Documentation: Personally identifiable information (PII))
Skills in:
- Validating data quality (for example, by using AWS Glue DataBrew and AWS Glue Data Quality)
- Identifying and mitigating sources of bias in data (for example, selection bias, measurement bias) by using AWS tools (for example, SageMaker Clarify)
- Preparing data to reduce prediction bias (for example, by using dataset splitting, shuffling, and augmentation)
- Configuring data to load into the model training resource (for example, Amazon EFS, Amazon FSx)
Domain 2: ML Model Development
Task Statement 2.1: Choose a modeling approach.
Knowledge of:
- Capabilities and appropriate uses of ML algorithms to solve business problems (AWS Documentation: Solving Business Problems with Amazon Machine Learning)
- How to use AWS artificial intelligence (AI) services (for example, Amazon Translate, Amazon Transcribe, Amazon Rekognition, Amazon Bedrock) to solve specific business problems (AWS Documentation: Transcribe, translate, and summarize)
- How to consider interpretability during model selection or algorithm selection (AWS Documentation: Machine learning model interpretability with AWS)
- SageMaker built-in algorithms and when to apply them (AWS Documentation: Built-in algorithms and pretrained models in Amazon SageMaker)
Skills in:
- Assessing available data and problem complexity to determine the feasibility of an ML solution
- Comparing and selecting appropriate ML models or algorithms to solve specific problems
- Choosing built-in algorithms, foundation models, and solution templates (for example, in SageMaker JumpStart and Amazon Bedrock)
- Selecting models or algorithms based on costs
- Selecting AI services to solve common business needs
Task Statement 2.2: Train and refine models.
Knowledge of:
- Elements in the training process (for example, epoch, steps, batch size) (AWS Documentation: Hyperparameters, Train and evaluate AWS DeepRacer models)
- Methods to reduce model training time (for example, early stopping, distributed training) (AWS Documentation: Stop Training Jobs Early)
- Factors that influence model size (AWS Documentation: Influence model responses with inference parameters)
- Methods to improve model performance (AWS Documentation: Debugging and improving model performance)
- Benefits of regularization techniques (for example, dropout, weight decay, L1 and L2) (AWS Documentation: Training Parameters)
- Hyperparameter tuning techniques (for example, random search, Bayesian optimization) (AWS Documentation: Understand the hyperparameter tuning strategies)
- Model hyperparameters and their effects on model performance (for example, number of trees in a tree-based model, number of layers in a neural network) (AWS Documentation: What is Hyperparameter Tuning?)
- Methods to integrate models that were built outside SageMaker into SageMaker
Skills in:
- Using SageMaker built-in algorithms and common ML libraries to develop ML models
- Using SageMaker script mode with SageMaker supported frameworks to train models (for example, TensorFlow, PyTorch)
- Using custom datasets to fine-tune pre-trained models (for example, Amazon Bedrock, SageMaker JumpStart)
- Performing hyperparameter tuning (for example, by using SageMaker automatic model tuning [AMT])
- Integrating automated hyperparameter optimization capabilities
- Preventing model overfitting, underfitting, and catastrophic forgetting (for example, by using regularization techniques, feature selection)
- Combining multiple training models to improve performance (for example, ensembling, stacking, boosting)
- Reducing model size (for example, by altering data types, pruning, updating feature selection, compression)
- Managing model versions for repeatability and audits (for example, by using the SageMaker Model Registry)
Task Statement 2.3: Analyze model performance.
Knowledge of:
- Model evaluation techniques and metrics (for example, confusion matrix, heat maps, F1 score, accuracy, precision, recall, Root Mean Square Error [RMSE], receiver operating characteristic [ROC], Area Under the ROC Curve [AUC]) (AWS Documentation: Define relevant evaluation metrics)
- Methods to create performance baselines (AWS Documentation: Create a model quality baseline)
- Methods to identify model overfitting and underfitting (AWS Documentation: Underfitting vs. Overfitting)
- Metrics available in SageMaker Clarify to gain insights into ML training data and models (AWS Documentation: Post-training Data and Model Bias Metrics)
- Convergence issues
Skills in:
- Selecting and interpreting evaluation metrics and detecting model bias
- Assessing tradeoffs between model performance, training time, and cost
- Performing reproducible experiments by using AWS services
- Comparing the performance of a shadow variant to the performance of a production variant
- Using SageMaker Clarify to interpret model outputs
- Using SageMaker Model Debugger to debug model convergence
Domain 3: Deployment and Orchestration of ML Workflows
Task Statement 3.1: Select deployment infrastructure based on existing architecture and requirements.
Knowledge of:
- Deployment best practices (for example, versioning, rollback strategies) (AWS Documentation: Deployment strategies, Redeploy and roll back a deployment with CodeDeploy)
- AWS deployment services (for example, SageMaker) (AWS Documentation: SageMaker)
- Methods to serve ML models in real time and in batches
- How to provision compute resources in production environments and test environments (for example, CPU, GPU) (AWS Documentation: Compute environments for AWS Batch)
- Model and endpoint requirements for deployment endpoints (for example, serverless endpoints, real-time endpoints, asynchronous endpoints, batch inference) (AWS Documentation: Deploy models with Amazon SageMaker Serverless Inference, Real-time inference)
- How to choose appropriate containers (for example, provided or customized) (AWS Documentation: Choosing an AWS container service)
- Methods to optimize models on edge devices (for example, SageMaker Neo) (AWS Documentation: Model performance optimization with SageMaker Neo)
Skills in:
- Evaluating performance, cost, and latency tradeoffs
- Choosing the appropriate compute environment for training and inference based on requirements (for example, GPU or CPU specifications, processor family, networking bandwidth)
- Selecting the correct deployment orchestrator (for example, Apache Airflow, SageMaker Pipelines)
- Selecting multi-model or multi-container deployments
- Selecting the correct deployment target (for example, SageMaker endpoints, Kubernetes, Amazon Elastic Container Service [Amazon ECS], Amazon Elastic Kubernetes Service [Amazon EKS], Lambda)
- Choosing model deployment strategies (for example, real time, batch)
Task Statement 3.2: Create and script infrastructure based on existing architecture and requirements.
Knowledge of:
- Difference between on-demand and provisioned resources (AWS Documentation: Choose the data stream capacity mode)
- How to compare scaling policies (AWS Documentation: Step and simple scaling policies for Amazon EC2 Auto Scaling)
- Tradeoffs and use cases of infrastructure as code (IaC) options (for example, AWS CloudFormation, AWS Cloud Development Kit [AWS CDK]) (AWS Documentation: What is the AWS CDK?)
- Containerization concepts and AWS container services
- How to use SageMaker endpoint auto scaling policies to meet scalability requirements (for example, based on demand, time) (AWS Documentation: Automatic scaling of Amazon SageMaker AI models)
Skills in:
- Applying best practices to enable maintainable, scalable, and cost-effective ML solutions (for example, automatic scaling on SageMaker endpoints, dynamically adding Spot Instances, by using Amazon EC2 instances, by using Lambda behind the endpoints)
- Automating the provisioning of compute resources, including communication between stacks (for example, by using CloudFormation, AWS CDK)
- Building and maintaining containers (for example, Amazon Elastic Container Registry [Amazon ECR], Amazon EKS, Amazon ECS, by using bring your own container [BYOC] with SageMaker)
- Configuring SageMaker endpoints within the VPC network
- Deploying and hosting models by using the SageMaker SDK
- Choosing specific metrics for auto scaling (for example, model latency, CPU utilization, invocations per instance)
Task Statement 3.3: Use automated orchestration tools to set up continuous integration and continuous delivery (CI/CD) pipelines.
Knowledge of:
- Capabilities and quotas for AWS CodePipeline, AWS CodeBuild, and AWS CodeDeploy (AWS Documentation: Quotas in AWS CodePipeline)
- Automation and integration of data ingestion with orchestration services (AWS Documentation: Automate data ingestion from AWS Data Exchange into Amazon S3)
- Version control systems and basic usage (for example, Git) (AWS Documentation: Using Git version control systems in AWS Glue)
- CI/CD principles and how they fit into ML workflows (AWS Documentation: CI/CD on AWS)
- Deployment strategies and rollback actions (for example, blue/green, canary, linear) (AWS Documentation: Deployment strategies)
- How code repositories and pipelines work together (AWS Documentation: CodePipeline pipeline structure reference)
Skills in:
- Configuring and troubleshooting CodeBuild, CodeDeploy, and CodePipeline, including stages
- Applying continuous deployment flow structures to invoke pipelines (for example, Gitflow, GitHub Flow)
- Using AWS services to automate orchestration (for example, to deploy ML models, automate model building)
- Configuring training and inference jobs (for example, by using Amazon EventBridge rules, SageMaker Pipelines, CodePipeline)
- Creating automated tests in CI/CD pipelines (for example, integration tests, unit tests, end-to-end tests)
- Building and integrating mechanisms to retrain models
Domain 4: ML Solution Monitoring, Maintenance, and Security
Task Statement 4.1: Monitor model inference.
Knowledge of:
- Drift in ML models (AWS Documentation: Feature attribution drift for models in production)
- Techniques to monitor data quality and model performance (AWS Documentation: Data and model quality monitoring, Data quality)
- Design principles for ML lenses relevant to monitoring (AWS Documentation: Well-Architected machine learning design principles)
Skills in:
- Monitoring models in production (for example, by using SageMaker Model Monitor)
- Monitoring workflows to detect anomalies or errors in data processing or model inference
- Detecting changes in the distribution of data that can affect model performance (for example, by using SageMaker Clarify)
- Monitoring model performance in production by using A/B testing
Task Statement 4.2: Monitor and optimize infrastructure and costs.
Knowledge of:
- Key performance metrics for ML infrastructure (for example, utilization, throughput, availability, scalability, fault tolerance) (AWS Documentation: CloudWatch metrics that are available for your instances)
- Monitoring and observability tools to troubleshoot latency and performance issues (for example, AWS X-Ray, Amazon CloudWatch Lambda Insights, Amazon CloudWatch Logs Insights) (AWS Documentation: Monitoring and troubleshooting Lambda functions)
- How to use AWS CloudTrail to log, monitor, and invoke re-training activities
- Differences between instance types and how they affect performance (for example, memory optimized, compute optimized, general purpose, inference optimized) (AWS Documentation: Amazon EC2 Instance types)
- Capabilities of cost analysis tools (for example, AWS Cost Explorer, AWS Billing and Cost Management, AWS Trusted Advisor) (AWS Documentation: AWS Cost Explorer)
- Cost tracking and allocation techniques (for example, resource tagging) (AWS Documentation: Organizing and tracking costs using AWS cost allocation tags)
Skills in:
- Configuring and using tools to troubleshoot and analyze resources (for example, CloudWatch Logs, CloudWatch alarms)
- Creating CloudTrail trails
- Setting up dashboards to monitor performance metrics (for example, by using Amazon QuickSight, CloudWatch dashboards)
- Monitoring infrastructure (for example, by using EventBridge events)
- Rightsizing instance families and sizes (for example, by using SageMaker Inference Recommender and AWS Compute Optimizer)
- Monitoring and resolving latency and scaling issues
- Preparing infrastructure for cost monitoring (for example, by applying a tagging strategy)
- Troubleshooting capacity concerns that involve cost and performance (for example, provisioned concurrency, service quotas, auto scaling)
- Optimizing costs and setting cost quotas by using appropriate cost management tools (for example, AWS Cost Explorer, AWS Trusted Advisor, AWS Budgets)
- Optimizing infrastructure costs by selecting purchasing options (for example, Spot Instances, On-Demand Instances, Reserved Instances, SageMaker Savings Plans)
Task Statement 4.3: Secure AWS resources.
Knowledge of:
- IAM roles, policies, and groups that control access to AWS services (for example, AWS Identity and Access Management [IAM], bucket policies, SageMaker Role Manager) (AWS Documentation: AWS Identity and Access Management for Amazon SageMaker AI)
- SageMaker security and compliance features (AWS Documentation: Configure security in Amazon SageMaker AI)
- Controls for network access to ML resources (AWS Documentation: Controlling Access to Amazon ML Resources -with IAM)
- Security best practices for CI/CD pipelines (AWS Documentation: Best practices for CI/CD pipelines)
Skills in:
- Configuring least privilege access to ML artifacts
- Configuring IAM policies and roles for users and applications that interact with ML systems
- Monitoring, auditing, and logging ML systems to ensure continued security and compliance
- Troubleshooting and debugging security issues
- Building VPCs, subnets, and security groups to securely isolate ML systems
– Practice Exams
Taking practice exams is one of the most effective ways to assess readiness and identify areas for improvement.
- Official AWS Practice Exams: AWS provides official practice exams that simulate the real test environment. These should be attempted multiple times to gauge understanding and adjust study plans accordingly.
- Third-Party Practice Exams: Supplementing with third-party mock exams can expose candidates to a wider variety of questions and testing patterns.
- Review Incorrect Answers: Carefully analyze incorrect responses and understand the reasoning behind correct choices. Creating summary notes or flashcards for weak areas will help reinforce learning.
– AWS Training and Certification Resources
AWS provides multiple training resources, both free and paid, to help candidates prepare for the exam effectively.
- AWS Training Courses: Enrolling in AWS instructor-led or online courses will provide structured learning and expert insights into ML concepts and AWS services.
- AWS Skill Builder: This platform offers self-paced learning modules, hands-on labs, and quizzes tailored to certification exams.
- AWS Certification Ramp-Up Guides: AWS provides official ramp-up guides that outline essential topics and recommended learning paths.
- AWS re:Invent Videos: Watching AWS re:Invent and Summit sessions related to machine learning can help candidates stay updated with the latest trends and best practices.
- Study Groups and Communities: Joining AWS certification study groups, forums, or online communities such as AWS Machine Learning LinkedIn groups or Reddit forums can be beneficial. Engaging with peers and discussing concepts enhances learning and problem-solving skills.
- Time Management Strategies: Practicing time management during mock exams is critical. Allocate specific time limits for each section to ensure all questions are attempted within the exam duration.
- Staying Updated: AWS frequently updates its services, so candidates should regularly check AWS blogs, release notes, and documentation to stay informed about the latest changes.
Conclusion
It’s crucial to reiterate that mastering the intricacies of AWS machine learning services is not merely about passing an exam; it’s about unlocking your potential to build transformative AI solutions that drive real-world impact. We’ve journeyed through the foundational principles of machine learning, dissected the functionalities of key services like Amazon SageMaker, and addressed the critical aspects of security, cost optimization, and deployment. Remember, this cheat sheet is a powerful tool, but it’s most effective when combined with hands-on experience, diligent study of AWS documentation, and consistent practice. The AWS cloud is a dynamic and ever-evolving landscape, demanding continuous learning and adaptation.
Embrace the challenge, delve deep into the resources provided, and leverage the vibrant AWS community to support your journey. By doing so, you’ll not only equip yourself to conquer the exam but also position yourself as a leading machine learning engineer, ready to innovate and shape the future of AI on the AWS platform. We wish you the best of luck in your preparation and look forward to celebrating your success.