Mastering Microsoft Fabric is no longer a luxury, but a necessity for any analytics professional aiming to leverage a truly unified, end-to-end data platform. This comprehensive DP-600: Implementing Analytics Solutions Using Microsoft Fabric cheat sheet is designed to demystify the intricacies of Fabric, from the foundational OneLake architecture and its seamless integration with services like Data Factory and Synapse to the powerful analytical capabilities of Power BI and Real-Time Analytics. We’ll dissect each domain of the exam, providing actionable insights into services like Dataflows Gen2, KQL databases, and Direct Lake, ensuring you’re not just familiar with the concepts, but proficient in their practical application.
This guide goes beyond surface-level definitions, delving into best practices for security, governance with Microsoft Purview, and performance optimization, equipping you with the knowledge and confidence to excel in the DP-600 exam and beyond. Let’s embark on this journey to transform you into a Microsoft Fabric expert.
Overview of Microsoft Fabric
Microsoft Fabric is a comprehensive, cloud-based analytics solution that unifies data integration, engineering, science, warehousing, real-time analytics, and business intelligence into a single platform. Designed to simplify complex data workflows, Fabric enables organizations to streamline analytics operations while leveraging a fully managed SaaS environment. Understanding the core components and functionalities of Fabric is crucial for those preparing for the Microsoft DP-600: Implementing Analytics Solutions Using Microsoft Fabric exam. This cheat sheet provides a structured overview of its key features, services, and best practices.
– Core Components of Microsoft Fabric
Fabric integrates multiple analytical workloads within a single, cohesive platform. At its heart is OneLake, a SaaS-based, unified data lake that serves as the central repository for all organizational data.
- OneLake – The Unified Data Lake
- Acts as a single, logical data lake for an entire organization.
- Eliminates data silos by offering a hierarchical, multi-workspace structure.
- Supports shortcuts to external data sources for seamless integration.
- Compatible with open data formats such as Delta Lake and Parquet.
- Data Integration: Data Factory in Fabric
- Enables robust ETL/ELT workflows through Data Pipelines and Dataflows Gen2.
- Supports native connectors for various Azure services, databases, APIs, and file-based storage.
- Incorporates Azure Data Factory (ADF) capabilities, including orchestration and automation.
- Synapse Data Engineering
- Built on Apache Spark, providing high-performance data transformation and preparation.
- Supports languages such as Python, Scala, and SQL.
- Allows for notebooks, Spark jobs, and Lakehouse tables to be created within OneLake.
- Synapse Data Science
- Facilitates machine learning model development, training, and deployment.
- Integrates with MLflow, AutoML, and Azure Machine Learning.
- Enables direct access to OneLake data for predictive analytics.
- Synapse Data Warehousing
- Provides a high-performance SQL-based warehouse for structured data storage and analysis.
- Utilizes Delta Lake tables for efficient data retrieval and transformations.
- Supports T-SQL, stored procedures, and indexing for optimized query performance.
- Synapse Real-Time Analytics
- Processes streaming data efficiently using Kusto Query Language (KQL).
- Supports event ingestion through Eventstreams.
- Enables real-time materialized views for fast querying and visualization.
- Power BI Integration
- Embedded deeply within Fabric for interactive dashboards and reports.
- Features Direct Lake mode for optimized performance.
- Supports Semantic Models and Datamarts for self-service data exploration.
– Key Advantages of Microsoft Fabric
Feature | Benefit |
---|---|
Unified Experience | Integrates multiple analytics services into one seamless platform. |
SaaS-Based Simplicity | Fully managed, reducing operational overhead and infrastructure costs. |
Open Data Formats | Supports Delta Lake and Parquet for enhanced interoperability. |
Enhanced Collaboration | OneLake enables seamless data access and sharing across teams. |
Governance & Security | Integrates with Microsoft Purview for data lineage, security, and compliance. |
Microsoft DP-600 Exam Cheat Sheet
Microsoft Fabric is a powerful, unified analytics platform to streamline data integration, engineering, science, warehousing, and real-time analytics. As organizations increasingly rely on data-driven insights, mastering Fabric’s capabilities is essential for professionals aiming to implement scalable and efficient analytics solutions. This cheat sheet provides a structured overview of Fabric’s key components, features, and best practices to help you confidently prepare for the DP-600 exam and excel in real-world analytics scenarios.
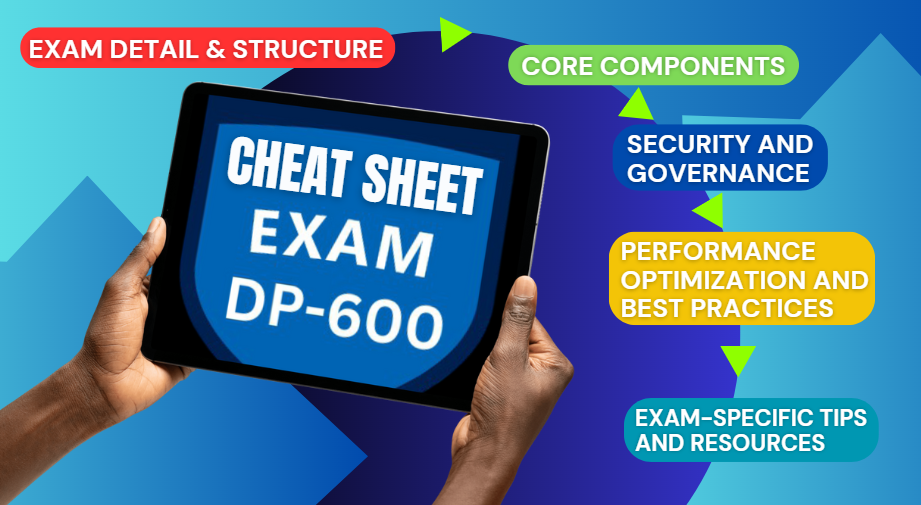
Microsoft DP-600 Exam Detail & Structure
As a candidate for Exam DP-600: Implementing Analytics Solutions Using Microsoft Fabric, you are expected to have expertise in designing, developing, and managing analytical assets, including semantic models, data warehouses, and lakehouses.
– Key Responsibilities
- Data Preparation & Enrichment: Transform and optimize data for analysis.
- Security & Maintenance: Ensure governance, compliance, and ongoing management of analytics assets.
- Semantic Model Implementation: Develop and manage structured data models to support business intelligence and reporting.
In this role, you will collaborate closely with stakeholders to gather business requirements and work alongside architects, analysts, engineers, and administrators to implement effective analytics solutions. Additionally, proficiency in querying and analyzing data using Structured Query Language (SQL), Kusto Query Language (KQL), and Data Analysis Expressions (DAX) is essential.
– Exam Details
The DP-600: Implementing Analytics Solutions Using Microsoft Fabric exam is a key certification for professionals seeking the Microsoft Certified: Fabric Analytics Engineer Associate credential. Candidates are given 100 minutes to complete the assessment, which is proctored and not open book. The exam may also include interactive components that require hands-on problem-solving.
This certification exam is available in multiple languages, including English, Japanese, Chinese (Simplified), German, French, Spanish, and Portuguese (Brazil), ensuring accessibility for a global audience. To successfully pass, candidates must achieve a minimum score of 700.
Core Components Deep Dive
Microsoft Fabric is a unified analytics platform that integrates various services to provide seamless data management, transformation, and analysis. Understanding the core components of Fabric is essential for professionals preparing for the DP-600 exam, as these components enable organizations to build, manage, and optimize their analytical solutions effectively. This section provides an in-depth exploration of Fabric’s key components, highlighting their functionalities, integrations, and best practices.
– Microsoft OneLake: The Unified Data Lake
1. Foundational Data Lake
OneLake serves as the central, SaaS-based data lake for an organization, simplifying data storage, management, and access. By providing a single copy of data, OneLake eliminates redundancy and promotes efficient data utilization across multiple analytical workloads. This unified storage system enables seamless collaboration, reduces storage costs, and enhances data governance.
2. Hierarchical Structure and Shortcuts
OneLake organizes data in a hierarchical manner, featuring workspaces and folders that structure data efficiently. A standout feature of OneLake is its shortcuts, which allow users to access data stored in different locations, including Azure Data Lake Storage (ADLS) Gen2, Amazon S3, and other Fabric workspaces, without duplicating data. This capability enhances accessibility and enables cross-platform data integration.
3. Security and Governance
Fabric integrates Microsoft Purview with OneLake, ensuring robust data governance, compliance, and security. Access control is managed via workspace roles and permissions, allowing organizations to define who can view or modify data. Additionally, OneLake supports data encryption to protect sensitive information and ensure regulatory compliance.
4. Integration with Fabric Components
OneLake seamlessly interacts with various Fabric components such as Data Factory, Synapse, and Power BI, providing a central repository for data processing and analysis. Open data formats like Delta Lake enhance compatibility and performance by enabling transactional capabilities within the lake.
– Data Factory in Fabric: Data Integration and Orchestration
1. Data Ingestion and Transformation
Data Factory facilitates data ingestion from diverse sources, including on-premises databases, cloud services, and SaaS applications. Users can design ETL/ELT workflows using pipelines and Dataflows Gen2, ensuring efficient data transformation before analytics processing.
2. Pipelines, Activities, and Data Flows
Data Factory pipelines consist of multiple activities, such as copying data, executing Spark notebooks, and running SQL scripts. These pipelines automate data movement and transformation, while Dataflows Gen2 provides a low-code/no-code approach for preparing data efficiently.
3. Orchestration and Scheduling
Fabric enables users to schedule and orchestrate data workflows through triggers and monitoring tools, ensuring that pipelines execute as planned. This automation minimizes manual intervention, optimizing the data preparation process.
– Synapse Data Engineering: Scalable Data Processing
1. Spark Pools and Notebooks
Fabric’s Synapse Data Engineering leverages Apache Spark to enable large-scale data processing. Users can write code in Python, Scala, and Spark SQL within interactive notebooks, facilitating data transformation and exploratory analysis.
2. Data Processing with Spark
Spark in Fabric enables efficient data cleaning, transformation, and aggregation within OneLake. Users can run Spark SQL queries directly on lakehouse tables, reducing the need for complex data movement.
3. Lakehouse Design and Implementation
Fabric adopts the Lakehouse architecture, combining the best of data lakes and warehouses. Organizations can define Lakehouse tables in OneLake, leveraging shortcuts to integrate external data seamlessly.
– Synapse Data Science: Machine Learning and AI
1. Machine Learning Workflows
Fabric provides an end-to-end machine learning (ML) environment, enabling users to prepare data, train models, and deploy them efficiently. It integrates with Azure Machine Learning to streamline ML lifecycle management.
2. MLflow and Experiment Tracking
MLflow enables model experiment tracking and versioning, allowing data scientists to compare different model iterations and optimize performance effectively.
3. AutoML and Notebooks for Data Science
AutoML accelerates ML model development by automating feature selection, training, and hyperparameter tuning. Additionally, notebooks facilitate exploratory data analysis and advanced ML workflows.
– Synapse Data Warehousing: Scalable and Efficient Data Storage
1. SQL Data Warehouse Capabilities
Fabric offers a high-performance, cloud-based data warehouse optimized for analytical workloads. It supports structured data storage, indexing, and partitioning, ensuring efficient query performance.
2. T-SQL Queries and Data Loading
Users can execute T-SQL queries for reporting and analysis, utilizing COPY INTO and PolyBase for seamless data loading into the warehouse.
3. Delta Tables and Dimensional Modeling
Fabric adopts Delta Lake tables, enabling ACID-compliant transactions within the warehouse. Users can implement star and snowflake schemas for efficient data modeling and reporting.
– Synapse Real-Time Analytics: Streaming Data Processing
1. Real-Time Data Ingestion and Processing
Fabric supports real-time data ingestion through Eventstreams, enabling organizations to process and analyze live data efficiently.
2. KQL Databases and Queries
The platform uses Kusto Query Language (KQL) for real-time analytics, allowing users to execute high-performance queries on streaming data.
3. Streaming Data Analysis and Materialized Views
Fabric’s real-time analytics capabilities support materialized views, optimizing query performance for live data monitoring and decision-making.
– Power BI in Fabric: Business Intelligence and Visualization
1. Power BI Integration
Power BI is deeply integrated into Fabric, allowing users to create interactive dashboards and reports directly on OneLake data.
2. Data Visualization and Reporting
Fabric enables users to leverage Power BI visuals, reports, and dashboards to gain actionable insights from their datasets.
3. Direct Lake Mode and Semantic Models
Direct Lake Mode enhances performance by enabling Power BI to query data directly from OneLake without the need for data import. Semantic models provide governed and structured data access for business intelligence applications.
4. Datamarts and Power BI Notebooks
Datamarts allow users to create self-service data warehouses, simplifying analytics workflows. Additionally, Power BI Notebooks offer an interactive environment for data exploration and transformation.
Security and Governance
In any data-driven organization, security and governance play a pivotal role in ensuring data protection, regulatory compliance, and controlled access. Microsoft Fabric incorporates robust security measures and governance frameworks to safeguard data integrity while allowing seamless collaboration across teams. This section explores the critical components of security and governance within the Fabric ecosystem.
– Access Control and Security
Effective access control mechanisms are fundamental in preventing unauthorized access and ensuring that users have appropriate permissions based on their roles.
1. Role-Based Access Control (RBAC) in Fabric
Fabric implements Role-Based Access Control (RBAC) across workspaces and data assets, ensuring that only authorized users can perform specific actions. The predefined roles—Administrator, Contributor, and Viewer—offer structured permission levels, while custom roles can be configured to meet more granular access requirements. Administrators can assign roles to users and groups, maintaining a secure yet flexible governance model.
2. Network Security and Data Encryption
Network security in Fabric is managed through various mechanisms, including virtual network integration and the use of private endpoints for secure connectivity. Data encryption is enforced both at rest and in transit, leveraging Microsoft-managed encryption by default, with the option to implement Customer-Managed Keys (CMK) for enhanced control over cryptographic processes.
3. Data Masking and Row-Level Security (RLS)
Protecting sensitive data is crucial in analytics environments. Data masking enables organizations to obscure sensitive information during querying and reporting, ensuring compliance with privacy regulations. Row-Level Security (RLS) further enhances access control by dynamically restricting data access based on user attributes or predefined roles. In Power BI semantic models, RLS ensures that users only see the data they are authorized to access, enhancing security across reports and dashboards.
4. Microsoft Purview Integration
Microsoft Purview plays a vital role in governing data across the Fabric ecosystem. By integrating Purview with Fabric, organizations can leverage advanced data discovery, classification, and cataloging capabilities. This integration helps in maintaining compliance and provides visibility into data lineage and ownership.
– Data Governance and Compliance
Establishing a strong governance framework is essential for ensuring data quality, compliance, and auditability within Microsoft Fabric.
1. Data Lineage and Data Cataloging
Understanding data flow and transformations is critical for maintaining data integrity. Fabric provides robust data lineage tracking, allowing users to trace data from its source to its consumption points. Additionally, the data catalog facilitates efficient data discovery and management, ensuring that assets are well-documented and accessible to authorized users. Microsoft Purview enhances these capabilities by offering automated lineage tracking and metadata management.
2. Data Quality and Validation
Maintaining high data quality is essential for accurate analytics and decision-making. Fabric provides tools for data validation, profiling, and monitoring, ensuring that data meets predefined quality standards. Dataflows Gen2 enables users to apply data transformation rules, automate validation processes, and improve overall data accuracy before analysis.
3. Compliance Requirements and Auditing
Organizations must comply with various regulatory standards such as GDPR, HIPAA, and SOC 2. Microsoft Fabric supports compliance by providing built-in auditing capabilities, enabling organizations to track user activity and data access. Audit logs record changes, user interactions, and security events, providing transparency and accountability in data governance.
4. Data Sensitivity Labels
Microsoft Purview’s sensitivity labels allow organizations to classify and protect data based on its confidentiality level. These labels help enforce security policies, prevent unauthorized sharing, and ensure compliance with industry regulations. Sensitivity labels are seamlessly integrated into Microsoft Fabric, enabling automated enforcement of data protection policies across all analytics workloads.
Performance Optimization and Best Practices
Ensuring optimal performance in Microsoft Fabric is crucial for building scalable and efficient analytics solutions. By following best practices and applying performance optimization techniques, organizations can enhance query execution speed, improve resource utilization, and maximize the efficiency of data processing across Fabric components. This section outlines strategies for performance tuning, best practices for data integration and warehousing, and real-time analytics optimization.
– Performance Tuning
Performance tuning in Microsoft Fabric involves optimizing queries, refining Spark job execution, and effectively managing data partitioning and distribution to enhance overall efficiency.
1. Query Optimization and Indexing
Efficient query execution is fundamental to performance in analytics workloads. Optimizing T-SQL and KQL queries involves using query plans to understand execution patterns and reduce unnecessary computations. Indexing strategies in Synapse Data Warehousing and KQL databases play a key role in accelerating query performance by enabling faster lookups and reducing scan times.
2. Spark Performance Tuning
Apache Spark is a core component of Synapse Data Engineering, and optimizing its performance requires careful consideration of job execution strategies. Partitioning and caching data can significantly improve processing speed by minimizing I/O operations. Additionally, adjusting Spark configurations such as memory allocation and parallelism settings helps maximize resource efficiency.
3. Data Partitioning and Distribution
Proper data partitioning and distribution strategies directly impact performance in OneLake and Synapse. Techniques such as hash partitioning and range partitioning ensure efficient data retrieval, reducing query execution time. Implementing best practices for partition management in OneLake improves performance by organizing data effectively.
4. OneLake Performance Best Practices
Optimizing OneLake storage performance involves structuring folders efficiently to avoid unnecessary complexity. Small files can degrade performance, so consolidating data into optimized file formats like Parquet and Delta Lake enhances read performance and reduces processing overhead.
– Best Practices for Data Integration
Data integration processes, including ETL (Extract, Transform, Load) and ELT (Extract, Load, Transform), must be optimized to ensure smooth data ingestion, transformation, and loading.
1. ETL/ELT Strategies
Understanding when to use ETL versus ELT is essential for efficient data movement. Best practices include designing streamlined data pipelines in Data Factory and leveraging Dataflows Gen2 for improved performance. Optimizing transformations at the right stage of the pipeline reduces processing delays and ensures data readiness for analysis.
2. Data Quality Management
Maintaining high data quality is vital for reliable analytics. Implementing robust data validation and cleansing techniques helps ensure accuracy and consistency. Establishing data quality rules within Data Factory ensures that only clean and validated data is ingested into analytical systems.
3. Error Handling and Monitoring
A proactive approach to error handling enhances data pipeline reliability. Implementing detailed logging mechanisms and setting up alerts allows teams to detect and resolve issues promptly. Monitoring pipeline executions and performance using built-in Fabric tools ensures continuous optimization and prevents system bottlenecks.
– Best Practices for Data Warehousing
Building efficient data warehouses requires strategic data modeling and optimized loading techniques to support analytical workloads.
1. Star and Snowflake Schema Design
Designing appropriate data models is essential for performance optimization. The star schema offers simplicity and faster query performance, while the snowflake schema provides normalization benefits. Choosing the right schema design depends on the complexity and performance requirements of the workload.
2. Fact and Dimension Table Optimization
Maintaining well-structured fact and dimension tables is crucial for query efficiency. Best practices include proper indexing, partitioning, and maintaining surrogate keys to improve join performance. Ensuring that fact tables store only necessary transactional data while dimensions are optimized for lookups enhances performance.
3. Incremental Data Loading
Incremental data loading reduces processing overhead by updating only new or modified records instead of reloading entire datasets. Implementing change data capture (CDC) and timestamp-based tracking ensures efficient data refresh cycles, minimizing processing time and improving query responsiveness.
– Best Practices for Real-Time Analytics
Optimizing real-time analytics in Microsoft Fabric involves tuning KQL queries, managing streaming data ingestion, and leveraging materialized views for enhanced query performance.
1. Optimizing KQL Queries
Efficient KQL queries improve real-time analytics performance. Best practices include using proper filtering techniques, leveraging functions, and indexing key columns for faster query execution. Materialized views play a crucial role in pre-aggregating data to enhance query responsiveness.
2. Streaming Data Ingestion
Handling high-volume streaming data efficiently requires robust ingestion strategies. Utilizing Eventstreams ensures real-time data processing with minimal latency. Configuring proper data retention policies and optimizing event processing logic helps maintain system stability.
3. Materialized Views Optimization
Materialized views provide precomputed query results for enhanced performance. Optimizing their creation involves carefully selecting aggregation levels, refreshing intervals, and indexing strategies to balance performance with storage considerations.
Exam-Specific Tips and Resources
Preparing for Exam DP-600: Implementing Analytics Solutions Using Microsoft Fabric requires a strategic approach that balances theoretical understanding with practical experience. This section provides an in-depth guide to help you navigate the exam effectively, including a detailed breakdown of its objectives, recommended study strategies, common pitfalls to avoid, and essential Microsoft resources.
– Understanding Exam Objectives
A crucial first step in preparing for the DP-600 exam is understanding its domains and objectives. The exam assesses your ability to design, implement, and manage analytics solutions using Microsoft Fabric. To maximize your study efforts, you should break down each domain and map it to relevant Fabric services and practical applications.
1. Detailed Breakdown of Domains
The DP-600 exam is structured around several key domains, each covering specific aspects of Fabric analytics:
1. Maintain a data analytics solution (25–30%)
Implement security and governance
- Implement workspace-level access controls
- Implement item-level access controls
- Implement row-level, column-level, object-level, and file-level access control
- Apply sensitivity labels to items
- Endorse items
Managing the analytics development lifecycle
- Implementing version control for a workspace (Microsoft Documentation: Version control, metadata search, and navigation)
- Creating and managing a Power BI Desktop project (.pbip) (Microsoft Documentation: Power BI Desktop projects (PREVIEW))
- Planning and implementing deployment pipelines (Microsoft Documentation: Planning the Deployment)
- Performing impact analysis of downstream dependencies from lakehouses, data warehouses, dataflows, and semantic models (Microsoft Documentation: Semantic model impact analysis)
- Deploying and managing semantic models by using the XMLA endpoint (Microsoft Documentation: Semantic model connectivity with the XMLA endpoint)
- Creating and updating reusable assets, including Power BI template (.pbit) files, Power BI data source (.pbids) files, and shared semantic models (Microsoft Documentation: Create and use report templates in Power BI Desktop, Semantic models in the Power BI service)
2. Learn how to Prepare data (45–50%)
Get data
- Create a data connection
- Discover data by using OneLake data hub and real-time hub
- Ingest or access data as needed
- Choose between a lakehouse, warehouse, or eventhouse
- Implement OneLake integration for eventhouse and semantic models
Transforming data
- Create views, functions, and stored procedures
- Enrich data by adding new columns or tables
- Implementing a star schema for a lakehouse or warehouse (Microsoft Documentation: Understand star schema and the importance for Power BI)
- Denormalizing data (Microsoft Documentation: Modeling for Performance)
- Aggregating data (Microsoft Documentation: User-defined aggregations)
- Merging or joining data (Microsoft Documentation: Merge queries (Power Query))
- Identifying and resolving duplicate data, missing data, or null values (Microsoft Documentation: Set up duplicate detection rules to keep your data clean)
- Convert column data types
- Filtering data
Query and analyze data
- Select, filter, and aggregate data by using the Visual Query Editor
- Select, filter, and aggregate data by using SQL
- Select, filter, and aggregate data by using KQL
3. Implement and manage semantic models (25–30%)
Designing and building semantic models
- Choosing a storage mode
- Implementing a star schema for a semantic model (Microsoft Documentation: Understand star schema and the importance for Power BI)
- Implementing relationships, such as bridge tables and many-to-many relationships (Microsoft Documentation: Many-to-many relationship guidance)
- Writing calculations that use DAX variables and functions, such as iterators, table filtering, windowing, and information functions (Microsoft Documentation: Use variables to improve your DAX formulas)
- Implementing calculation groups, dynamic strings, and field parameters (Microsoft Documentation: Calculation groups)
- Identify use cases for and configure large semantic model storage format (Microsoft Documentation: Datasets larger than 10 GB in Power BI Premium)
- Designing and building composite models (Microsoft Documentation: Use composite models in Power BI Desktop)
Optimizing enterprise-scale semantic models
- Implementing performance improvements in queries and report visuals (Microsoft Documentation: Optimization guide for Power BI)
- Improving DAX performance (Microsoft Documentation: Performance Tuning DAX )
- Configure Direct Lake, including default fallback and refresh behavior
- Implement incremental refresh for semantic models (Microsoft Documentation: Incremental refresh and real-time data for semantic models)
Understanding these domains will allow you to focus on the most critical areas of the exam while identifying your strengths and weaknesses in each topic.
2. Mapping Objectives to Fabric Services
Each exam objective is directly tied to a specific Fabric service or feature. Mapping these objectives will help you connect theoretical knowledge to practical implementation. Below are some key mappings:
- Data Ingestion → Implemented using Data Factory pipelines
- Data Transformation → Managed through Dataflows Gen2 and Spark notebooks
- Data Storage and Management → Handled within OneLake, Lakehouse, and Delta tables
- Security and Compliance → Governed by Microsoft Purview and sensitivity labels
- Data Analysis and Reporting → Performed using Power BI, KQL, and Synapse analytics tools
3. Deciphering Microsoft’s Exam Language
Microsoft exams often use specific terminology that requires careful interpretation. Here are some key action words and what they typically imply:
- “Implement” → You should know how to configure and deploy a feature in a real-world scenario.
- “Manage” → You must understand administrative tasks, maintenance, and monitoring of Fabric components.
- “Optimize” → You should be able to improve performance, efficiency, and scalability of analytics solutions.
- “Troubleshoot” → You need to identify and resolve issues in Fabric workloads, queries, or infrastructure.
4. Analyzing Case Studies and Scenario-Based Questions
Microsoft exams often feature case studies that assess your ability to apply concepts in real-world business scenarios. When tackling these questions:
- Carefully read the scenario to identify business and technical requirements.
- Determine the most efficient and scalable solution based on Fabric capabilities.
- Look for keywords that hint at specific Fabric services or best practices.
- Use the process of elimination to discard incorrect answer choices.
– Study Strategies
A structured and effective study plan can significantly improve your chances of passing the DP-600 exam. Below are recommended strategies:
1. Effective Study Planning
- Create a study schedule that allows you to dedicate focused time to each exam domain.
- Use a mix of learning methods: official documentation, hands-on practice, video tutorials, and practice exams.
- Focus on hands-on experience—the exam tests practical knowledge as much as theory.
2. Practice Exams and Study Materials
- Take official Microsoft practice exams to familiarize yourself with the exam format.
- Use reputable resources such as Microsoft Learn modules.
- Review exam-specific study guides and documentation to reinforce key concepts.
3. Online Communities and Discussion Forums
Engaging in online communities can provide valuable insights and help resolve doubts. Recommended platforms include:
- Microsoft Tech Community – Discussions on Fabric implementation and exam strategies.
- Stack Overflow – Troubleshooting technical issues.
- Reddit (r/Azure and r/PowerBI) – Peer insights and experiences.
– Common Exam Pitfalls
Understanding common mistakes can help you avoid unnecessary errors on exam day.
1. Mistakes to Avoid
- Overlooking security and governance – RBAC, Purview, and RLS are frequently tested but often neglected.
- Ignoring OneLake concepts – Fabric’s unified data lake architecture is central to multiple exam domains.
- Skipping performance optimization – Be familiar with query optimization, indexing, and partitioning techniques.
2. Time Management Tips
- Don’t spend too much time on one question – If unsure, mark it for review and return later.
- Prioritize easier questions first to maximize your score before tackling difficult ones.
- Watch out for “trick questions” that test real-world best practices rather than textbook knowledge.
– Microsoft Resources
Microsoft provides a wealth of official learning resources that can help you build a strong foundation in Microsoft Fabric while preparing for the DP-600 certification exam. Leveraging these resources effectively can make a significant difference in your understanding and exam performance.
1. Official Documentation and Learning Paths
One of the most valuable study tools is Microsoft’s official documentation, which provides in-depth explanations, step-by-step guides, and best practices for using Microsoft Fabric’s components. The Microsoft Fabric Documentation covers everything from data ingestion, transformation, and storage to security, governance, and real-time analytics. It is an essential reference for reinforcing conceptual knowledge and ensuring you understand how Fabric services function in real-world scenarios.
Additionally, Microsoft’s official DP-600 learning path on Microsoft Learn offers a structured way to study the exam objectives. These learning paths contain interactive lessons, knowledge checks, and exercises designed to help you grasp key topics. Since these modules align directly with the exam syllabus, they are particularly useful for those who prefer a guided study approach.
2. Microsoft Learn Modules
Microsoft Learn provides self-paced, hands-on training that is invaluable for practical experience. These modules cover topics such as data ingestion and transformation using Data Factory, managing Lakehouses and Warehouses, optimizing Power BI semantic models, and implementing security within Fabric. Completing these modules will allow you to apply theoretical knowledge to real-world scenarios, helping you develop a deeper understanding of Microsoft Fabric’s architecture and workflows.
A major advantage of Microsoft Learn is its interactive format, which often includes sandbox environments where you can practice deploying Fabric components without needing a separate Azure subscription. This hands-on experience is crucial for grasping data pipeline orchestration, query optimization, and security configurations, which are all key topics on the DP-600 exam.
3. Hands-on Labs and Sandboxes
One of the best ways to reinforce learning is through practical, hands-on experience. Microsoft offers Fabric trial environments and Azure sandboxes, which allow you to experiment with different Fabric services in a real-world setting. Using these environments, you can practice building data pipelines, optimizing queries, configuring security roles, and designing efficient semantic models—all skills that will be tested on the exam.
In addition, Microsoft provides hands-on labs and guided exercises that walk you through common analytics scenarios, such as loading data into OneLake, configuring Power BI Direct Lake connections, and managing Synapse Real-Time Analytics workloads. These labs are an excellent way to gain confidence in implementing Fabric solutions before taking the actual exam.
4. Instructor-led training and Community Support
For learners who prefer structured guidance, Microsoft offers instructor-led training courses for DP-600 preparation. These courses, led by certified trainers, provide deeper insights into Fabric’s features, best practices, and exam-relevant scenarios. Attending these sessions can be particularly beneficial for professionals who want to clarify doubts and receive expert feedback on their approach to solving Fabric-related challenges.
Apart from formal training, engaging with the Microsoft community can be extremely beneficial. Platforms like Microsoft Tech Community, Stack Overflow, LinkedIn groups, and Reddit (r/Azure, r/PowerBI) are great places to discuss exam strategies, troubleshoot issues, and stay updated with the latest Fabric developments. Many professionals share their exam experiences, study materials, and practical tips, making these forums valuable resources for candidates preparing for DP-600.
Conclusion
This cheat sheet has aimed to distill the essential knowledge, best practices, and exam-specific insights needed to navigate the complexities of Fabric and excel in the exam. By focusing on core components, security and governance, performance optimization, and understanding the exam objectives, you’re now equipped to confidently approach the DP-600. Remember that practical application and hands-on experience are crucial; leverage Microsoft Learn modules, practice exams, and engage with online communities to reinforce your learning. Embrace the power of Fabric to transform data into actionable insights, and let this certification be a testament to your expertise in building modern analytics solutions. We encourage you to put this knowledge into practice, tackle the exam with confidence, and share your experiences to inspire others in their journey.