In today’s data-driven world, artificial intelligence (AI) and machine learning (ML) are no longer futuristic concepts but essential tools for businesses across all sectors. As the demand for skilled AI practitioners grows, the AWS Certified AI Practitioner certification has emerged as a crucial validation of foundational knowledge in leveraging Amazon Web Services (AWS) for AI/ML solutions. This certification caters to both technical and business professionals, bridging the gap between understanding AI/ML concepts and their practical application within the AWS ecosystem. Whether you’re aiming to enhance your career prospects, gain a competitive edge, or simply solidify your grasp of AWS AI/ML services, this AWS Certified AI Practitioner Cheat Sheet will serve as your invaluable companion.
We’ll explore the core ML principles, dissect key AWS AI/ML services like Amazon SageMaker, Rekognition, and Comprehend, explore practical use cases, and address critical aspects of security, compliance, and MLOps. This guide goes beyond surface-level information, providing you with a detailed overview of essential terminology, algorithms, and best practices to confidently tackle the exam and apply your knowledge in real-world scenarios. Let’s begin on this journey to demystify AWS AI/ML and pave your way to certification success.
AWS AI Certification Benefits
In an era where artificial intelligence (AI) and machine learning (ML) are reshaping industries, obtaining the AWS Certified AI Practitioner credential offers a significant advantage. This certification validates foundational AI/ML expertise within the AWS ecosystem, making it a valuable asset for both technical and non-technical professionals.
- Validation of Foundational AI/ML Knowledge on AWS
- Earning this certification demonstrates a strong understanding of core AI/ML principles and their practical applications within AWS. It signifies your ability to identify suitable AWS AI/ML services for specific business needs while reinforcing your knowledge of machine learning, deep learning, and AI-driven decision-making.
- Career Advancement and Increased Employability
- The demand for AI/ML professionals continues to rise, and this certification enhances your career prospects by showcasing validated skills. It positions you as a strong candidate for roles such as AI/ML Specialist, Cloud Solution Architect, Data Scientist (AWS-focused), or Business Analyst with AI/ML expertise. Additionally, it demonstrates a commitment to staying current with emerging technologies, making you a valuable asset in a competitive job market.
- Enhanced Understanding of AWS AI/ML Services
- Through this certification, you gain practical knowledge of essential AWS AI/ML services such as Amazon SageMaker, Rekognition, Comprehend, Lex, and Polly. You develop a deep understanding of their functionalities, differences, and appropriate use cases, enabling you to efficiently integrate these tools into real-world solutions.
- Improved Communication with Technical Teams
- For business professionals, this certification acts as a bridge between business requirements and technical implementation. It allows for more effective collaboration with data scientists, ML engineers, and AI developers by providing a foundational understanding of AI/ML capabilities and limitations. This ensures better communication and alignment between business objectives and AI-driven strategies.
- Competitive Advantage
- Holding the AWS Certified AI Practitioner certification sets you apart from others in the field by demonstrating a proactive approach to learning and professional development. It serves as tangible proof of your expertise in AI/ML, reinforcing your credibility and positioning you as a forward-thinking professional in an evolving industry.
- Personal and Professional Growth
- The process of preparing for and earning this certification enhances critical thinking, problem-solving, and analytical skills. It fosters a sense of achievement, boosts confidence in your AI/ML abilities, and strengthens your ability to adapt to technological advancements, making you a more versatile professional.
Purpose of AWS Certified AI Practitioner Cheat Sheet
The AWS Certified AI Practitioner Cheat Sheet is designed as a concise yet comprehensive resource to streamline your exam preparation. It presents key AI, ML, and AWS-related concepts in an organized and structured manner, making it an essential tool for both conceptual clarity and quick revision.
- Structured Overview of Key Concepts
- This cheat sheet offers a well-organized breakdown of the fundamental concepts covered in the AWS Certified AI Practitioner exam. It simplifies complex topics into digestible sections, ensuring an intuitive learning experience. The logical arrangement of information guides you through core AI/ML principles and their application within AWS, helping you grasp the material more effectively.
- Quick Reference for Exam Preparation
- Serving as a readily accessible resource, this cheat sheet allows for rapid revision of essential topics. Whether you need a quick refresher on algorithms, AI models, or AWS services, or are conducting a last-minute review before the exam, this document helps reinforce critical areas of knowledge.
- Efficient and Targeted Study Aid
- Aligned with the AWS Certified AI Practitioner exam objectives, this cheat sheet ensures that you focus on the most relevant material. It highlights key terms, definitions, and real-world applications, acting as a study roadmap to navigate the vast AWS documentation and prioritize crucial exam content.
- Bridging Theoretical and Practical Knowledge
- Beyond theoretical understanding, this cheat sheet connects AI/ML principles with their practical implementation in AWS. It includes examples of how AWS AI/ML services are used to solve real-world challenges, helping you comprehend not just how these technologies work, but also why they are applied in specific scenarios.
- Reinforcing Learning and Retention
- This cheat sheet serves as a reinforcement tool to consolidate knowledge acquired from other study methods. It complements practice exams, study guides, and hands-on experience, helping you retain critical concepts more effectively.
- Clarifying Essential Terminology
- Understanding the terminology used in AI/ML and AWS is crucial for the exam. This cheat sheet defines and explains key terms and industry jargon, while also providing context on how they are applied in real-world AI/ML scenarios.
AWS Certified AI Practitioner Cheat Sheet
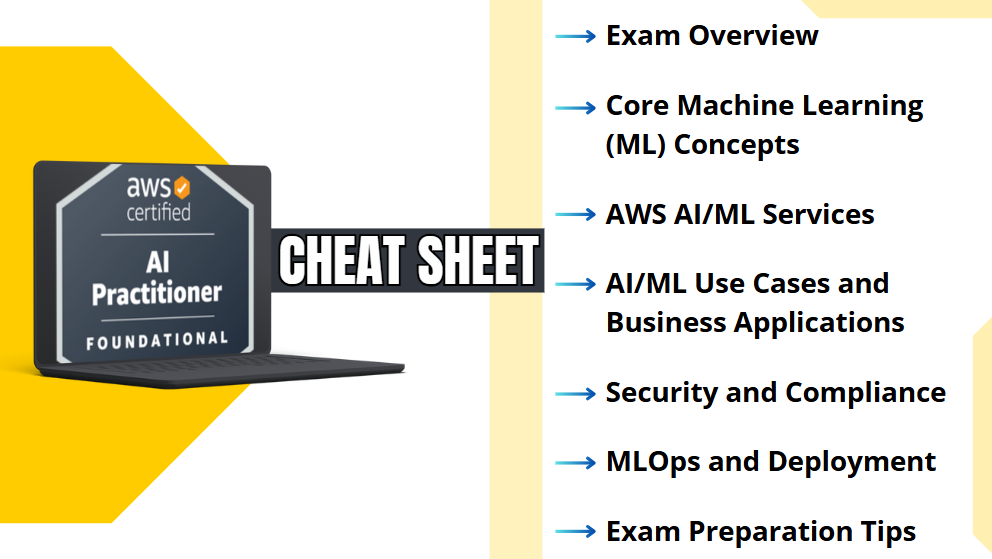
AWS Certified AI Practitioner Exam Overview
The AWS Certified AI Practitioner credential validates foundational knowledge in artificial intelligence (AI), machine learning (ML), and generative AI, along with their real-world applications. This certification enhances your professional credibility, strengthens your competitive advantage, and positions you for career advancement and increased earning potential.
The exam is designed for individuals who can effectively demonstrate a broad understanding of AI/ML and generative AI technologies, along with the AWS services and tools that support them. Unlike role-specific certifications, this credential focuses on general AI/ML expertise applicable across various industries and job functions.
Key Areas of Knowledge Assessed
The exam evaluates a candidate’s ability to:
- Understand core AI, ML, and generative AI concepts, methodologies, and strategies, both in general and within the AWS ecosystem.
- Identify appropriate AI/ML and generative AI technologies to address specific business challenges and use cases.
- Determine the correct AI/ML approaches for different scenarios and apply best practices.
- Promote responsible AI usage, ensuring ethical and effective implementation.
Target Candidate Profile
Ideal candidates for this certification should have up to six months of exposure to AI/ML technologies on AWS. While they may not build AI/ML solutions from the ground up, they should be familiar with AI/ML applications and use cases within AWS environments.
Recommended AWS Knowledge
Candidates should have a basic understanding of AWS services and cloud fundamentals, including:
- Core AWS services, such as Amazon EC2, Amazon S3, AWS Lambda, and Amazon SageMaker, along with their primary use cases.
- The AWS shared responsibility model, particularly regarding security and compliance.
- AWS Identity and Access Management (IAM) for controlling access and securing AWS resources.
- AWS global infrastructure concepts, including Regions, Availability Zones, and edge locations.
- AWS pricing models, ensuring a clear understanding of cost-effective service usage.
Exam Details
The AWS Certified AI Practitioner Exam is a foundational-level certification designed for individuals with familiarity in AI/ML technologies on AWS, even if they do not actively develop AI/ML solutions. The exam consists of 65 questions, with a duration of 90 minutes, and is scored on a scaled range of 100–1,000, requiring a minimum passing score of 700.
This certification is ideal for professionals such as business analysts, IT support specialists, marketing professionals, product or project managers, line-of-business or IT managers, and sales professionals who seek to enhance their AI/ML knowledge within the AWS ecosystem. Candidates can take the exam at a Pearson VUE testing center or opt for an online proctored exam. The exam is available in English, Japanese, Korean, Portuguese (Brazil), and Simplified Chinese.
Core Machine Learning (ML) Concepts
Machine Learning (ML) is the foundation of modern artificial intelligence, enabling systems to learn from data, identify patterns, and make decisions with minimal human intervention. ML is broadly categorized into supervised, unsupervised, and reinforcement learning, each serving distinct purposes based on the availability of labeled data and the desired outcome.
– Fundamental ML Terminology
1. Supervised Learning
Supervised learning relies on labeled data, where the model learns from input-output pairs. It maps features (input variables) to labels (output values) by identifying relationships between them. The goal is to generalize this learned mapping to unseen data. Supervised learning is divided into two main types:
- Classification: The task of predicting categorical labels. For example, an email filtering system classifies messages as “spam” or “not spam.”
- Common Algorithms: Logistic Regression, Decision Trees, Random Forest, Support Vector Machines (SVMs), Naïve Bayes.
- Regression: Used when the output is a continuous value, such as predicting house prices or stock market trends.
- Common Algorithms: Linear Regression, Polynomial Regression, Ridge Regression.
2. Unsupervised Learning
Unlike supervised learning, unsupervised learning works with unlabeled data, where the algorithm detects underlying patterns and structures. It is commonly used for clustering, anomaly detection, and dimensionality reduction.
Key types of unsupervised learning include:
- Clustering: Organizes data into meaningful groups without predefined labels. It is widely used in customer segmentation, fraud detection, and social network analysis.
- Examples: K-Means, Hierarchical Clustering, DBSCAN.
- Dimensionality Reduction: Reduces the number of input variables while retaining the most important information, improving computational efficiency.
- Examples: Principal Component Analysis (PCA), t-SNE, Autoencoders.
3. Reinforcement Learning (RL)
Reinforcement Learning (RL) is a unique ML paradigm where an agent interacts with an environment, learning through trial and error by maximizing cumulative rewards. Instead of learning from static datasets, the agent makes sequential decisions and receives feedback to refine its strategy.
Key Components of RL:
- Agent: The decision-maker (e.g., an AI playing a game).
- Environment: The system in which the agent operates.
- Actions: The possible moves the agent can make.
- Rewards: Feedback signals that reinforce good decisions.
Applications of RL:
- Game AI (e.g., AlphaGo, OpenAI Five).
- Robotics (e.g., autonomous navigation, industrial automation).
- Self-driving cars (e.g., optimizing braking and acceleration).
4. Training, Validation, and Test Data
A dataset is typically divided into three subsets to ensure effective learning and evaluation:
- Training Data: Used to train the ML model by adjusting parameters based on patterns in the data.
- Validation Data: Helps fine-tune hyperparameters to prevent overfitting.
- Test Data: Used to assess the final model’s performance on unseen data.
5. Features, Labels, and Models
- Features: The input variables that influence the prediction (e.g., age, income, temperature).
- Labels: The output variable in supervised learning (e.g., “loan approved” or “loan rejected”).
- Model: A mathematical function that maps inputs (features) to outputs (labels).
6. Overfitting vs. Underfitting
- Overfitting: The model memorizes training data instead of learning patterns, leading to poor generalization on unseen data.
- Underfitting: The model is too simplistic and fails to capture the underlying trends in data, resulting in poor accuracy.
7. Bias-Variance Tradeoff
- Bias: The error due to overly simplistic assumptions (causing underfitting).
- Variance: The error due to excessive sensitivity to training data (causing overfitting).
- Goal: Find an optimal balance between bias and variance to improve predictive performance.
8. Performance Metrics for ML Models
To evaluate an ML model, various metrics are used depending on the problem type:
- Accuracy: Measures the overall correctness of predictions.
- Precision: The proportion of correctly predicted positive instances out of all predicted positives.
- Recall (Sensitivity): The proportion of actual positives that were correctly identified.
- F1-Score: The harmonic mean of precision and recall, balancing both metrics.
- AUC (Area Under the ROC Curve): Evaluates a classifier’s ability to distinguish between classes.
9. Loss Functions
Loss functions measure how well a model’s predictions match actual outcomes.
- Mean Squared Error (MSE): Used in regression, calculating the average squared difference between actual and predicted values.
- Cross-Entropy Loss: Used in classification problems to measure the divergence between predicted probabilities and actual labels.
10. Optimization Algorithms
Optimization techniques adjust model parameters to minimize loss functions:
- Gradient Descent: Iteratively adjusts weights to minimize the loss function.
- Adam (Adaptive Moment Estimation): An advanced optimization technique that adapts learning rates for faster convergence.
– Common ML Algorithms
1. Linear Regression
A fundamental regression algorithm that models the relationship between a dependent variable and one or more independent variables.
y=mx+b
where:
- y is the predicted value,
- m is the slope,
- x is the input variable,
- b is the intercept.
2. Logistic Regression
A classification algorithm that estimates the probability of a binary outcome using the sigmoid function.
3. Decision Trees
A tree-like model where decisions are made based on feature values. It is simple to interpret but prone to overfitting.
4. Random Forests
An ensemble learning technique combining multiple decision trees to improve accuracy and robustness.
5. Gradient Boosted Trees (XGBoost, LightGBM, CatBoost)
Boosting algorithms that iteratively refine weak models to create a strong predictive model.
6. K-Means Clustering
An unsupervised algorithm that partitions data into K clusters, grouping similar data points.
7. Principal Component Analysis (PCA)
A dimensionality reduction technique that extracts the most important features while preserving variance in the dataset.
8. Neural Networks and Activation Functions
Artificial Neural Networks (ANNs) mimic the human brain, with layers of interconnected neurons.
- ReLU (Rectified Linear Unit): f(x)=max(0,x)
- Sigmoid: f(x)=1+e−x1
- Tanh: f(x)=ex+e−xex−e−x
– Data Preprocessing
1. Data Cleaning
- Handling Missing Values: Use imputation techniques (mean, median, mode) or remove missing entries.
- Handling Outliers: Detect and remove extreme values to prevent biased predictions.
2. Feature Scaling
- Normalization: Scales values to a range of [0,1].
- Standardization: Rescales features to have a mean of 0 and standard deviation of 1.
3. Feature Engineering
Creating new meaningful features from existing data, such as extracting time-based patterns from timestamps.
4. Data Splitting
Common train-validation-test splits: 70/15/15 or 80/10/10.
AWS AI/ML Services: A Comprehensive Guide
Amazon Web Services (AWS) offers a diverse range of artificial intelligence (AI) and machine learning (ML) services to help businesses automate tasks, gain valuable insights, and enhance user experiences. These services cater to both developers and data scientists, providing powerful tools for computer vision, natural language processing (NLP), speech recognition, recommendation systems, forecasting, and more.
AWS AI/ML services eliminate the complexities of building, training, and deploying models from scratch, allowing organizations to focus on innovation and business applications. Whether you’re a beginner exploring ML or an enterprise scaling AI solutions, AWS provides fully managed AI services, pre-trained models, and custom ML tools to meet your needs. Below is a detailed breakdown of AWS AI/ML services, highlighting their capabilities, key features, and real-world applications.
1. Amazon SageMaker
Amazon SageMaker is a fully managed machine learning (ML) service designed to help developers and data scientists build, train, and deploy ML models at scale. It provides an end-to-end machine learning development environment, enabling businesses to automate workflows, manage models, and improve productivity.
Traditionally, machine learning requires significant computational power, extensive data preparation, and expertise in model tuning. SageMaker simplifies this by offering a comprehensive set of ML tools, reducing infrastructure complexity and making it easier to scale models from prototype to production.
– Key Components
- SageMaker Studio – A web-based integrated development environment (IDE) for ML, offering a unified interface for data preprocessing, model training, and deployment.
- SageMaker Notebooks – Fully managed Jupyter notebooks, eliminating the need for manual setup.
- SageMaker Experiments – Tracks, organizes, and compares different ML experiments.
- SageMaker Autopilot – Automates model selection and hyperparameter tuning, enabling users to create ML models without deep ML expertise.
- Built-in Algorithms – Optimized algorithms for tasks such as classification, regression, clustering, and recommendation systems.
- Bring Your Own Model (BYOM) – Supports custom models built using frameworks like TensorFlow, PyTorch, and MXNet.
- SageMaker Training & Hosting – Provides scalable infrastructure for training and deploying models efficiently.
- SageMaker Neo – Optimizes models for various hardware platforms, improving inference efficiency.
- SageMaker Ground Truth – Automates data labeling using machine learning-assisted workflows.
- SageMaker Clarify – Helps detect bias in ML models, ensuring fairness and transparency.
- SageMaker Feature Store – A centralized repository for storing and managing ML features.
- SageMaker Pipelines – Automates the ML workflow, integrating CI/CD (Continuous Integration/Continuous Deployment) practices.
– Use Cases
- Developing AI-driven applications such as fraud detection, predictive analytics, and automation.
- Enhancing operational efficiency by automating ML workflows.
- Improving model performance through built-in hyperparameter tuning and optimization.
- Ensuring model fairness and compliance by detecting bias in ML algorithms.
2. Amazon Rekognition
Amazon Rekognition is a computer vision service that provides advanced image and video analysis capabilities. It helps businesses detect objects, people, text, and activities in images and videos, enabling applications such as facial recognition, content moderation, and security surveillance.
This service leverages deep learning algorithms to deliver highly accurate image analysis, making it an ideal solution for industries like retail, security, healthcare, and media.
– Key Features
- Object and Scene Detection – Identifies objects, animals, vehicles, and scenes in an image.
- Facial Recognition – Detects age, emotions, facial landmarks, and attributes (e.g., sunglasses, beard).
- Optical Character Recognition (OCR) – Extracts text from images and videos.
- Custom Labels – Enables businesses to train custom ML models for specialized image recognition tasks.
- Video Analysis – Detects activities and unsafe content in real-time video streams.
– Use Cases
- Security & authentication – Facial recognition for access control and identity verification.
- Content moderation – Detects and removes explicit or inappropriate content in user-generated media.
- Retail & marketing – Automated image tagging and cataloging.
- Media analytics – Identifies celebrities, logos, and brand elements in videos.
3. Amazon Comprehend
Amazon Comprehend is an NLP (Natural Language Processing) service that helps businesses extract meaningful insights from text. It can analyze large volumes of unstructured data, including customer feedback, emails, and social media posts, providing actionable intelligence for decision-making.
– Key Features
- Sentiment Analysis – Determines whether text conveys positive, negative, neutral, or mixed sentiment.
- Entity Recognition – Identifies names, organizations, locations, and key terms within a document.
- Topic Modeling – Groups text into relevant topics based on machine learning analysis.
- Custom Entity Recognition & Classification – Allows businesses to train models to recognize industry-specific terms.
– Use Cases
- Customer sentiment analysis – Extracting opinions from reviews and surveys.
- Automated document classification – Tagging and organizing documents for faster retrieval.
- Social media monitoring – Tracking brand mentions and customer feedback in real time.
4. Amazon Translate
Amazon Translate is a neural machine translation service that provides real-time and batch translation for over 75 languages. It is designed to maintain context, tone, and accuracy across translations, making it an essential tool for global businesses.
– Key Features
- Real-time and batch translation for documents, chat applications, and websites.
- Custom Terminology – Enables businesses to define industry-specific translations.
– Use Cases
- Website localization – Translating content for global audiences.
- Multilingual customer support – Automating real-time chat translations.
5. Amazon Transcribe
Amazon Transcribe is a speech-to-text service that converts audio into highly accurate text. It supports various languages and dialects, making it ideal for call centers, media, and accessibility applications.
– Key Features
- Speaker identification – Recognizes different speakers in a conversation.
- Custom Vocabularies – Improves transcription accuracy for domain-specific terms.
– Use Cases
- Meeting transcription – Automatically converts meetings and interviews into text.
- Call center analytics – Extracts insights from customer interactions.
6. Amazon Lex
Amazon Lex is a fully managed AI service for building conversational interfaces using text and voice. It enables developers to create chatbots and virtual assistants that can interact naturally with users through speech or text-based conversations.
Amazon Lex is powered by the same deep learning technology as Amazon Alexa, making it highly scalable and capable of understanding complex conversations. It integrates seamlessly with other AWS services, such as AWS Lambda, Amazon Connect, and Amazon Polly, to create intelligent and interactive applications.
– Key Features
- Automatic Speech Recognition (ASR) – Converts spoken language into text, making it useful for voice-enabled applications.
- Natural Language Understanding (NLU) – Identifies user intent and extracts key information (slots) from conversations.
- Multi-turn Conversations – Supports dynamic, context-aware dialogues that guide users step by step.
- Seamless AWS Integration – Works with AWS Lambda, Amazon Connect (for call centers), and Amazon DynamoDB.
- Multi-platform Deployment – Deploy chatbots on web, mobile apps, and messaging platforms (e.g., Facebook Messenger, Slack).
– Use Cases
- Customer service automation – Reduces call center workload by handling common inquiries through chatbots.
- Voice-activated applications – Powers voice interfaces for smart home devices and mobile apps.
- Interactive FAQ chatbots – Automates responses to frequently asked questions on websites and apps.
7. Amazon Polly
Amazon Polly is a text-to-speech (TTS) service that converts text into lifelike speech using advanced AI models. It enables businesses to build interactive voice applications, such as audiobooks, virtual assistants, and accessibility tools.
Amazon Polly offers multiple voices, languages, and speech customization options, including Neural Text-to-Speech (NTTS) for high-quality, natural-sounding speech synthesis.
– Key Features
- Multiple Languages & Voices – Supports over 60 voices in 30+ languages, including male and female variations.
- Neural Text-to-Speech (NTTS) – Produces more natural, human-like speech.
- Custom Lexicons – Allows customized pronunciation for specific words or phrases.
- SSML Support (Speech Synthesis Markup Language) – Fine-tunes speech with pauses, emphasis, and pitch adjustments.
– Use Cases
- Audiobook and podcast narration – Converts text into high-quality audio content.
- Interactive voice response (IVR) systems – Used in call centers for automated customer service.
- Assistive technology – Enhances accessibility for visually impaired users.
8. Amazon Personalize
Amazon Personalize is a real-time recommendation engine that allows businesses to deliver personalized content to users based on their preferences and interactions. It uses machine learning algorithms to analyze user behavior and generate tailored recommendations for e-commerce, media, and marketing applications. Amazon Personalize is designed for businesses that want to offer Netflix-style recommendations without needing an in-house ML team.
– Key Features
- User-Item Interaction Tracking – Learns from customer behavior to enhance recommendations.
- Real-time Recommendations – Provides instant, personalized content suggestions.
- Customizable ML Models – Supports predefined and custom recommendation models.
- Easy Integration – Works with applications via simple API calls.
– Use Cases
- E-commerce product recommendations – Enhances shopping experiences by suggesting relevant products.
- Media content recommendations – Suggests movies, shows, or music based on user preferences.
- Personalized email marketing – Delivers customized promotions and offers to users.
9. Amazon Forecast
Amazon Forecast is a time-series forecasting service that uses machine learning to predict future trends and demands with high accuracy. It helps businesses make data-driven decisions for inventory management, financial planning, and resource allocation.
Traditional forecasting methods require manual analysis and domain expertise, but Amazon Forecast automates the entire process using deep learning algorithms, including the DeepAR+ algorithm.
– Key Features
- Automated Time-Series Forecasting – Uses ML-powered predictive models to analyze trends.
- DeepAR+ Algorithm – Provides highly accurate predictions for complex datasets.
- Related Time Series (RTS) – Enhances accuracy by incorporating external data (e.g., holidays, weather).
- Customizable Forecast Models – Supports domain-specific forecasting for various industries.
– Use Cases
- Retail demand forecasting – Predicts sales trends to optimize inventory.
- Financial forecasting – Estimates revenue, expenses, and market fluctuations.
- Supply chain optimization – Reduces waste and ensures adequate stock levels.
10. AWS DeepLens
AWS DeepLens is a computer vision-enabled camera designed for edge-based deep learning inference. It allows developers to build, train, and deploy ML models directly on the device, making it ideal for real-time image processing and object detection. DeepLens is fully programmable and integrates seamlessly with Amazon SageMaker, AWS Lambda, and AWS IoT, enabling AI-powered edge computing.
– Key Features
- Built-in Camera for ML Inference – Enables local object detection and image classification.
- Seamless AWS Integration – Connects with AWS SageMaker and Lambda for AI model deployment.
- Supports Popular ML Frameworks – Works with TensorFlow, MXNet, and PyTorch.
- Edge Processing – Runs inference locally, reducing cloud processing costs.
– Use Cases
- Smart surveillance – Identifies suspicious activities in security systems.
- Retail analytics – Tracks customer behavior and product interaction.
- Healthcare applications – Assists in medical image analysis.
11. AWS Inferentia & Trainium
AWS Inferentia and Trainium are custom-designed AWS chips optimized for high-performance deep learning inference and training. These chips provide cost-efficient, low-latency AI model deployment, enabling businesses to run complex ML workloads at scale.
Inferentia is designed for inference workloads, while Trainium is optimized for training large deep learning models. Both provide a significant performance boost over traditional GPUs for AI workloads.
– Key Features
- High Throughput & Low Latency – Accelerates AI inference tasks while reducing costs.
- Optimized for Deep Learning Frameworks – Supports TensorFlow, PyTorch, and MXNet.
- Scalability – Enables businesses to train and deploy models more efficiently.
– Use Cases
- Large-scale AI model training – Speeds up deep learning model training for NLP, vision, and speech recognition.
- Real-time AI inference – Processes AI predictions with lower costs compared to GPUs.
- Enterprise AI applications – Supports AI-driven chatbots, recommendations, and analytics.
AI/ML Use Cases and Business Applications
AI and ML are transforming industries by enabling businesses to automate processes, gain insights from data, and enhance decision-making. From customer experience personalization to fraud detection and predictive analytics, AI/ML applications drive efficiency, innovation, and competitive advantage. This section explores common use cases, the business value of AI/ML, and key ethical considerations in implementation.
1. Common Use Cases
Artificial Intelligence (AI) and Machine Learning (ML) are revolutionizing industries by providing intelligent solutions to complex business challenges. Below are some of the most impactful use cases demonstrating how AI/ML is driving innovation and efficiency across various sectors.
– Customer Churn Prediction
Customer retention is critical for business growth, and AI/ML enables organizations to predict and mitigate customer churn effectively. By analyzing customer interactions, demographics, and behavioral patterns, ML models identify at-risk customers, allowing companies to implement proactive retention strategies such as personalized offers or enhanced customer support.
AWS Tools: Amazon SageMaker, Amazon Forecast.
– Fraud Detection
AI-powered fraud detection systems enhance security by identifying anomalies in financial transactions and user activities. Machine learning models analyze transaction history, IP addresses, and login patterns to detect suspicious behavior in real time, reducing financial risks and improving security. Additionally, AI can be used for document fraud detection by verifying authenticity through advanced image recognition.
AWS Tools: Amazon SageMaker, Amazon Rekognition.
– Image Recognition for Product Identification
Organizations can leverage AI-based image recognition to streamline product identification for inventory management, quality control, and visual search applications. Computer vision models analyze product images, detect defects, and automate sorting processes, reducing reliance on manual inspections and improving operational efficiency.
AWS Tools: Amazon Rekognition, SageMaker Custom Labels.
– Sentiment Analysis for Customer Feedback
Understanding customer sentiment is crucial for brand management and service enhancement. AI-driven sentiment analysis enables businesses to process customer reviews, social media comments, and survey responses to gauge public opinion. These insights help organizations refine marketing strategies and improve customer engagement.
AWS Tools: Amazon Comprehend.
– Personalized Recommendations for E-commerce
E-commerce platforms uses AI-driven recommendation engines to enhance user experience by suggesting products based on browsing history, purchase behavior, and preferences. Personalized recommendations increase customer engagement, drive conversions, and foster brand loyalty.
AWS Tools: Amazon Personalize.
– Time Series Forecasting for Inventory Management
Accurate demand forecasting is essential for optimizing inventory levels and avoiding stockouts or overstocking. AI-powered predictive analytics analyze historical sales data, seasonal trends, and external factors such as market fluctuations to provide accurate demand predictions.
AWS Tools: Amazon Forecast.
– Chatbots for Customer Service
AI-driven chatbots revolutionize customer support by providing instant responses to common inquiries. These chatbots enhance customer experience by offering 24/7 assistance, reducing wait times, and improving resolution efficiency. They can be integrated with multiple communication channels, including websites, mobile apps, and social media.
AWS Tools: Amazon Lex.
– Medical Image Analysis
AI is transforming healthcare by assisting in the detection and diagnosis of diseases through medical image analysis. Deep learning models analyze X-rays, MRIs, and CT scans to identify anomalies, aiding radiologists in faster and more accurate diagnostics, ultimately improving patient outcomes.
AWS Tools: Amazon SageMaker, Amazon Rekognition.
2. Business Value of AI/ML
The adoption of AI/ML delivers significant business value by driving automation, enhancing decision-making, and reducing costs. Below are the key benefits AI/ML brings to modern enterprises:
– Increased Efficiency and Automation
AI-powered automation streamlines repetitive tasks, reducing manual effort and improving workforce productivity. Whether it is processing large datasets, automating customer inquiries, or optimizing supply chain logistics, AI enhances operational efficiency, allowing businesses to focus on strategic initiatives.
– Improved Customer Experience
AI enables businesses to deliver personalized services and support, improving customer satisfaction. AI-driven chatbots, personalized product recommendations, and sentiment analysis ensure that customers receive timely and relevant assistance, leading to better engagement and loyalty.
– Data-Driven Decision Making
By leveraging AI for data analytics, businesses can extract valuable insights from structured and unstructured data. AI algorithms identify trends, predict outcomes, and support informed decision-making, giving organizations a competitive edge in the market.
– Cost Reduction
AI-driven optimization minimizes costs by automating labor-intensive processes, preventing fraud, and improving resource allocation. AI-based forecasting ensures that businesses avoid unnecessary expenses related to inventory mismanagement or inefficient logistics planning.
– Innovation and Competitive Advantage
AI fosters innovation by enabling businesses to develop cutting-edge solutions and improve existing products. From AI-powered healthcare diagnostics to autonomous vehicles, AI is driving breakthroughs across industries, allowing companies to stay ahead of the competition.
3. Ethical Considerations
As AI adoption grows, ethical concerns must be addressed to ensure fairness, transparency, and accountability in AI applications.
– Bias in AI/ML Models
AI models can inherit biases from training data, leading to unfair outcomes. Organizations must employ techniques such as bias detection and mitigation to ensure equitable AI applications across all demographics.
AWS Tools: Amazon SageMaker Clarify.
– Data Privacy and Security
AI-driven systems process vast amounts of sensitive data, making security a top priority. Organizations must implement stringent data protection measures, such as encryption, access controls, and compliance with global privacy regulations like GDPR and CCPA.
AWS Tools: IAM (Identity and Access Management), KMS (Key Management Service), S3 Bucket Policies.
– Explainability and Transparency
AI models often function as black boxes, making it challenging to interpret their decisions. Ensuring transparency in AI predictions helps build trust and accountability. Techniques like model explainability and interpretable AI enhance understanding and confidence in AI-driven decisions.
– Responsible AI Development
Organizations should prioritize ethical AI development by considering societal impacts, ensuring regulatory compliance, and aligning AI goals with human-centric values. Establishing ethical AI governance frameworks fosters responsible AI usage and mitigates potential risks.
Security and Compliance
Security and compliance are critical aspects of deploying AI/ML solutions in the cloud. Organizations handling sensitive data must ensure that their AI/ML workflows adhere to the highest security standards and regulatory requirements. AWS provides a comprehensive set of security features, tools, and compliance programs to safeguard AI/ML workloads. This includes
1. Data Security in AWS AI/ML
Ensuring data security is a critical aspect of deploying AI/ML workloads on AWS. AWS provides a robust security framework with various tools and services designed to protect sensitive data, prevent unauthorized access, and ensure compliance with industry standards.
– IAM Roles and Policies
AWS Identity and Access Management (IAM) enables fine-grained control over user and service access to AWS resources. Implementing the principle of least privilege ensures that only authorized users and services have the necessary permissions.
- IAM roles facilitate secure access to AWS AI/ML services without the need for long-term credentials.
- Granular IAM policies define access control based on specific conditions, such as resource type, IP address, and time of access.
- Multi-factor authentication (MFA) adds an extra layer of security for user authentication.
– Encryption at Rest and in Transit
Encryption safeguards data from unauthorized access, both when stored and during transmission. AWS offers encryption mechanisms to protect AI/ML workloads.
- Data at Rest: AWS Key Management Service (KMS) enables encryption of stored data in Amazon S3, Amazon RDS, and other services.
- Data in Transit: Secure Sockets Layer/Transport Layer Security (SSL/TLS) ensures encrypted communication between clients and AWS AI/ML services.
- SageMaker encrypts notebook storage and ML endpoints to enhance data confidentiality.
– VPC Security
A Virtual Private Cloud (VPC) enhances network security by providing an isolated environment for AI/ML applications.
- Security groups and Network ACLs control inbound and outbound traffic.
- VPC endpoints allow private communication with AWS AI/ML services, eliminating exposure to the public internet.
- AWS PrivateLink enables secure service-to-service communication within the AWS ecosystem.
– S3 Bucket Policies
Amazon S3 bucket policies enforce strict access controls to prevent unauthorized data exposure.
- IAM-based access restrictions ensure that only authorized roles can access data.
- Server-side encryption (SSE) protects data stored in S3 using AWS KMS-managed keys.
- Enabling versioning and access logging enhances data integrity and auditability.
2. Compliance Standards
AWS AI/ML services adhere to various global compliance standards to ensure regulatory compliance for businesses operating in highly regulated industries.
– GDPR (General Data Protection Regulation)
AWS provides tools and best practices to help organizations comply with GDPR regulations, which mandate strong data protection measures.
- Data anonymization and pseudonymization techniques minimize privacy risks.
- Organizations must define responsibilities between data controllers and processors to ensure GDPR compliance.
- AWS services, such as Amazon Macie, help in detecting and protecting sensitive data.
– HIPAA (Health Insurance Portability and Accountability Act)
AWS offers HIPAA-compliant services to support organizations handling Protected Health Information (PHI).
- AWS provides a Business Associate Agreement (BAA) to ensure compliance obligations are met.
- Encryption and access controls help protect PHI stored in AWS AI/ML applications.
- Amazon Comprehend Medical facilitates secure processing of health-related text data.
– PCI DSS (Payment Card Industry Data Security Standard)
For organizations processing payment transactions, AWS provides services that align with PCI DSS standards.
- AWS-hosted applications must implement strong access controls and encryption for cardholder data.
- The shared responsibility model ensures that AWS secures the infrastructure, while customers configure application-level security.
- AWS Config and AWS Security Hub help monitor and maintain PCI DSS compliance.
– AWS Compliance Programs
AWS maintains a broad set of industry certifications and compliance reports to help organizations meet regulatory requirements.
- Certifications include SOC 1, SOC 2, ISO 27001, FedRAMP, and others.
- AWS Artifact provides access to compliance reports and documentation.
- AWS Well-Architected Framework ensures security best practices are followed.
3. Access Control
Access control mechanisms protect AI/ML resources from unauthorized access, ensuring only authenticated users and services interact with sensitive data.
– Principle of Least Privilege
Following the least privilege principle minimizes security risks by granting only the permissions necessary to perform specific tasks.
- Role-based access control (RBAC) ensures users can only access resources required for their roles.
- AWS Organizations allow centralized management of access policies across multiple accounts.
- AWS Secrets Manager securely stores and rotates access credentials to reduce the risk of exposure.
– AWS KMS (Key Management Service)
AWS KMS provides centralized management of encryption keys, ensuring secure handling of sensitive information.
- User-defined policies control access to encryption keys.
- Automatic key rotation enhances security without manual intervention.
- Integration with AWS AI/ML services enables seamless encryption of data.
– Auditing and Logging
Regular monitoring and auditing of access logs help detect security threats and ensure compliance.
- AWS CloudTrail: Logs API calls and user activities for auditability.
- Amazon CloudWatch: Monitors security events and triggers alerts.
- AWS Security Hub: Provides a unified view of security alerts across AWS accounts.
MLOps and Deployment
Deploying and managing machine learning models in production requires robust strategies to ensure efficiency, reliability, and scalability. MLOps (Machine Learning Operations) combines machine learning, DevOps, and data engineering practices to streamline the lifecycle of ML models—from development and training to deployment and monitoring. This section explores different deployment strategies, monitoring techniques, CI/CD pipelines for automation, and best practices for managing ML models at scale.
1. Model Deployment Strategies
– Real-Time Inference
Real-time inference enables models to generate instant predictions via API endpoints, making it ideal for applications requiring low latency and high throughput. For example, recommendation engines, fraud detection systems, and chatbots depend on real-time inference to provide immediate responses. AWS offers Amazon SageMaker Hosting, which allows models to be deployed as endpoints that handle live inference requests. Users can configure instance types, autoscaling options, and endpoint settings to optimize performance.
– Batch Inference
Batch inference is suitable for scenarios where real-time responses are not necessary, such as processing large datasets for customer insights, financial forecasting, or medical image analysis. Instead of serving predictions one at a time, batch inference processes multiple records simultaneously. AWS provides Amazon SageMaker Batch Transform, which enables organizations to run inference jobs on large datasets stored in Amazon S3. Understanding data input formats, batch size configurations, and resource optimization is key to ensuring efficiency.
– Serverless Inference (AWS Lambda)
For use cases with infrequent or unpredictable workloads, deploying ML models as serverless functions using AWS Lambda provides cost efficiency and scalability. AWS Lambda integrates with SageMaker Runtime API, allowing models to make predictions without provisioning or managing servers. Developers can configure Lambda functions with memory allocation and execution time limits, ensuring optimal performance for lightweight ML workloads.
– Endpoint Management
Managing model endpoints involves handling updates, versioning, and rollback strategies to ensure production stability. Amazon SageMaker endpoints support advanced deployment techniques like A/B testing, canary deployments, and shadow deployments, allowing businesses to test new models in production environments while minimizing risk. Continuous endpoint monitoring ensures models remain responsive and performant under varying workloads.
2. Model Monitoring
– Data Drift Detection
Over time, input data distributions can change, impacting model performance. Amazon SageMaker Model Monitor continuously analyzes incoming data and compares it with the model’s training data to detect data drift. Setting up automated alerts helps organizations take corrective actions, such as retraining the model with updated data.
– Model Performance Monitoring
Tracking model performance metrics, such as accuracy, precision, recall, and F1-score, is essential for maintaining reliability in production. AWS provides Amazon CloudWatch to monitor real-time performance metrics and identify potential degradation. By integrating CloudWatch with SageMaker, organizations can establish dashboards for visualizing performance trends.
– Alerting and Notifications
Timely intervention is critical when issues arise in production. Setting up alerts using Amazon CloudWatch Alarms and Amazon Simple Notification Service (SNS) ensures that data scientists and engineers receive notifications when performance drops, anomalies occur, or data drift exceeds acceptable thresholds.
3. CI/CD for ML
– Automated Training and Deployment
Automating the ML lifecycle improves efficiency and reduces errors. AWS CodePipeline and CodeBuild facilitate automated training, testing, and deployment workflows for ML models. By integrating SageMaker with CI/CD pipelines, organizations can ensure seamless model updates while maintaining high reliability.
– Version Control for Models
Tracking model versions is crucial for reproducibility and auditing. Tools like Git are commonly used for code and data versioning, while Amazon S3 stores model artifacts. Amazon SageMaker Experiments helps track training runs, model versions, and hyperparameter configurations, making it easier to roll back to previous models if needed.
– Orchestration Tools
Managing complex ML workflows requires orchestration tools like AWS Step Functions and SageMaker Pipelines. These tools enable seamless coordination of multiple ML tasks, including data preprocessing, training, validation, and deployment, ensuring smooth end-to-end execution.
4. Containerization (Docker)
– Deployment of Models in Containers
Containerization simplifies ML model deployment by packaging dependencies, configurations, and code into a portable environment. Docker containers are widely used for deploying ML models on Amazon SageMaker, Amazon ECS, and Amazon EKS. AWS Elastic Container Registry (ECR) securely stores Docker images for easy integration with deployment pipelines.
– Container Orchestration
For large-scale ML applications, container orchestration plays a crucial role in managing workloads efficiently. Amazon SageMaker integrates with Kubernetes-based orchestration systems like Amazon EKS, allowing enterprises to scale ML model deployments dynamically.
5. Infrastructure as Code (IaC)
– AWS CloudFormation and AWS CDK
Infrastructure as Code (IaC) ensures consistency across environments by defining infrastructure components in code. AWS CloudFormation and AWS CDK (Cloud Development Kit) allow organizations to provision and manage ML infrastructure—including SageMaker endpoints, training jobs, and data pipelines—programmatically. This improves scalability, maintainability, and repeatability of ML deployments.
– Reproducibility Across Environments
Using IaC helps prevent configuration drift by ensuring that ML infrastructure remains consistent across development, staging, and production environments. This enhances reproducibility, making it easier for teams to debug and deploy ML models confidently.
AWS Certified AI Practitioner Exam Preparation Tips
Preparing for the AWS Certified AI Practitioner exam requires a strategic approach that balances theoretical knowledge with practical experience. This certification assesses your understanding of AWS AI/ML services, their applications, and fundamental machine learning concepts. To ensure success, it’s essential to leverage official resources, practice extensively, and develop a structured study plan. Below are key strategies to help you confidently approach the exam and improve your chances of passing.
1. Practice Questions
– Use AWS Sample Questions and Practice Exams
Before diving deep into studying, familiarize yourself with the official AWS sample questions to understand the exam format and question types. AWS provides sample questions that reflect real exam scenarios, helping you gauge the level of complexity. Additionally, investing in reputable practice exams can simulate the real exam experience, highlight weak areas, and enhance your ability to manage time effectively.
Rather than simply memorizing answers, focus on understanding the reasoning behind correct responses. AWS exams often test conceptual clarity and practical application, so knowing why an answer is correct will help you tackle variations of the same concept.
– Analyze Incorrect Answers
Learning from mistakes is just as important as getting the right answers. When reviewing practice questions, take the time to understand why incorrect choices were wrong and identify recurring mistakes. Are you misunderstanding AWS service capabilities? Are you struggling with specific machine learning concepts? By recognizing patterns in errors, you can prioritize those areas for deeper study.
2. Hands-on Experience
– Work with AWS AI/ML Services in the AWS Management Console
AWS certifications emphasize practical application, so hands-on experience is crucial. If you haven’t already, create an AWS Free Tier account and explore AI/ML services like Amazon SageMaker, Rekognition, Comprehend, Personalize, and Forecast. By deploying a machine learning model, analyzing images using Rekognition, or extracting insights from text with Comprehend, you’ll gain firsthand experience that reinforces theoretical knowledge.
– Focus on Practical Scenarios
The exam tests not just what these services do but when and why to use them. Try to relate each AWS service to real-world applications. For example:
- When should you use Amazon Personalize instead of Amazon Forecast?
- How does Amazon Rekognition handle image classification differently from a custom-trained SageMaker model?
Understanding these distinctions will help you answer scenario-based questions confidently.
3. AWS Documentation
1. Refer to Official AWS Topics and Documentation
AWS documentation is one of the most reliable and comprehensive resources available. It provides in-depth details about each service, best practices, and common use cases. The topics are:
Domain 1: Fundamentals of AI and ML
Task Statement 1.1: Explain basic AI concepts and terminologies.
Objectives:
- Define basic AI terms (for example, AI, ML, deep learning, neural networks, computer vision, natural language processing [NLP], model, algorithm, training and inferencing, bias, fairness, fit, large language model [LLM]). (AWS Documentation: What Is Artificial Intelligence (AI)?)
- Describe the similarities and differences between AI, ML, and deep learning. (AWS Documentation: Difference Between Machine Learning and Deep Learning, AI and Machine Learning)
- Describe various types of inferencing (for example, batch, real-time). (AWS Documentation: Real-time inference, Run batch inference jobs)
- Describe the different types of data in AI models (for example, labeled and unlabeled, tabular, time-series, image, text, structured and unstructured). (AWS Documentation: Structured Data and Unstructured Data)
- Describe supervised learning, unsupervised learning, and reinforcement learning. (AWS Documentation: Supervised and Unsupervised Learning, What is Reinforcement Learning?)
Task Statement 1.2: Identify practical use cases for AI.
Objectives:
- Recognize applications where AI/ML can provide value (for example, assist human decision making, solution scalability, automation).
- Determine when AI/ML solutions are not appropriate (for example, costbenefit analyses, situations when a specific outcome is needed instead of a prediction).
- Select the appropriate ML techniques for specific use cases (for example, regression, classification, clustering). (AWS Documentation: Types of ML Models, Types of Algorithms)
- Identify examples of real-world AI applications (for example, computer vision, NLP, speech recognition, recommendation systems, fraud detection, forecasting). (AWS Documentation: Amazon Computer Vision)
- Explain the capabilities of AWS managed AI/ML services (for example, SageMaker, Amazon Transcribe, Amazon Translate, Amazon Comprehend, Amazon Lex, Amazon Polly). (AWS Documentation: AWS AI services)
Task Statement 1.3: Describe the ML development lifecycle.
Objectives:
- Describe components of an ML pipeline (for example, data collection, exploratory data analysis [EDA], data pre-processing, feature engineering, model training, hyperparameter tuning, evaluation, deployment, monitoring). (AWS Documentation: ML lifecycle phase – Data processing)
- Understand sources of ML models (for example, open source pre-trained models, training custom models). (AWS Documentation: Built-in algorithms and pretrained models, Model training)
- Describe methods to use a model in production (for example, managed API service, self-hosted API). (AWS Documentation: Model deployment options in Amazon SageMaker AI)
- Identify relevant AWS services and features for each stage of an ML pipeline (for example, SageMaker, Amazon SageMaker Data Wrangler, Amazon SageMaker Feature Store, Amazon SageMaker Model Monitor). (AWS Documentation: Amazon SageMaker)
- Understand fundamental concepts of ML operations (MLOps) (for example, experimentation, repeatable processes, scalable systems, managing technical debt, achieving production readiness, model monitoring, model re-training).
- Understand model performance metrics (for example, accuracy, Area Under the ROC Curve [AUC], F1 score) and business metrics (for example, cost per user, development costs, customer feedback, return on investment [ROI]) to evaluate ML models.
Domain 2: Fundamentals of Generative AI
Task Statement 2.1: Explain the basic concepts of generative AI.
Objectives:
- Understand foundational generative AI concepts (for example, tokens, chunking, embeddings, vectors, prompt engineering, transformer-based LLMs, foundation models, multi-modal models, diffusion models). (AWS Documentation: Foundation Models)
- Identify potential use cases for generative AI models (for example, image, video, and audio generation; summarization; chatbots; translation; code generation; customer service agents; search; recommendation engines). (AWS Documentation: Generative AI)
- Describe the foundation model lifecycle (for example, data selection, model selection, pre-training, fine-tuning, evaluation, deployment, feedback). (AWS Documentation: Foundation models and hyperparameters for fine-tuning)
Task Statement 2.2: Understand the capabilities and limitations of generative AI for solving business problems.
Objectives:
- Describe the advantages of generative AI (for example, adaptability, responsiveness, simplicity). (AWS Documentation: Generative AI)
- Identify disadvantages of generative AI solutions (for example, hallucinations, interpretability, inaccuracy, nondeterminism).
- Understand various factors to select appropriate generative AI models (for example, model types, performance requirements, capabilities, constraints, compliance). (AWS Documentation: Choosing a generative AI service)
- Determine business value and metrics for generative AI applications (for example, cross-domain performance, efficiency, conversion rate, average revenue per user, accuracy, customer lifetime value). (AWS Documentation: Delivering Business Value through Generative AI)
Task Statement 2.3: Describe AWS infrastructure and technologies for building generative AI applications.
Objectives:
- Identify AWS services and features to develop generative AI applications (for example, Amazon SageMaker JumpStart; Amazon Bedrock; PartyRock, an Amazon Bedrock Playground; Amazon Q). (AWS Documentation: Amazon SageMaker AI, Amazon Bedrock)
- Describe the advantages of using AWS generative AI services to build applications (for example, accessibility, lower barrier to entry, efficiency, cost-effectiveness, speed to market, ability to meet business objectives).
- Understand the benefits of AWS infrastructure for generative AI applications (for example, security, compliance, responsibility, safety). (AWS Documentation: Security perspective: Compliance and assurance of AI systems)
- Understand cost tradeoffs of AWS generative AI services (for example, responsiveness, availability, redundancy, performance, regional coverage, token-based pricing, provision throughput, custom models).
Domain 3: Applications of Foundation Models
Task Statement 3.1: Describe design considerations for applications that use foundation models.
Objectives:
- Identify selection criteria to choose pre-trained models (for example, cost, modality, latency, multi-lingual, model size, model complexity, customization, input/output length).
- Understand the effect of inference parameters on model responses (for example, temperature, input/output length). (AWS Documentation: Influence response generation with inference parameters, Inference request parameters and response fields)
- Define Retrieval Augmented Generation (RAG) and describe its business applications (for example, Amazon Bedrock, knowledge base). (AWS Documentation: RAG (Retrieval-Augmented Generation), Amazon Bedrock Knowledge Bases)
- Identify AWS services that help store embeddings within vector databases (for example, Amazon OpenSearch Service, Amazon Aurora, Amazon Neptune, Amazon DocumentDB [with MongoDB compatibility], Amazon RDS for PostgreSQL). (AWS Documentation: Vector Database)
- Explain the cost tradeoffs of various approaches to foundation model customization (for example, pre-training, fine-tuning, in-context learning, RAG). (AWS Documentation: Tradeoff analysis on custom versus pre-trained models)
- Understand the role of agents in multi-step tasks (for example, Agents for Amazon Bedrock). (AWS Documentation: Automate tasks in your application using AI agents)
Task Statement 3.2: Choose effective prompt engineering techniques.
Objectives:
- Describe the concepts and constructs of prompt engineering (for example, context, instruction, negative prompts, model latent space). (AWS Documentation: Prompt Engineering)
- Understand techniques for prompt engineering (for example, chain-ofthought, zero-shot, single-shot, few-shot, prompt templates). (AWS Documentation: Prompt templates and examples for Amazon Bedrock text models)
- Understand the benefits and best practices for prompt engineering (for example, response quality improvement, experimentation, guardrails, discovery, specificity and concision, using multiple comments).
- Define potential risks and limitations of prompt engineering (for example, exposure, poisoning, hijacking, jailbreaking). (AWS Documentation: Common prompt injection attacks)
Task Statement 3.3: Describe the training and fine-tuning process for foundation models.
Objectives:
- Describe the key elements of training a foundation model (for example, pre-training, fine-tuning, continuous pre-training). (AWS Documentation: Prepare your training datasets for fine-tuning and continued pre-training)
- Define methods for fine-tuning a foundation model (for example, instruction tuning, adapting models for specific domains, transfer learning, continuous pre-training). (AWS Documentation: Fine-tune a large language model (LLM) using domain adaptation)
- Describe how to prepare data to fine-tune a foundation model (for example, data curation, governance, size, labeling, representativeness, reinforcement learning from human feedback [RLHF]). (AWS Documentation: Fine-tune foundation models)
Task Statement 3.4: Describe methods to evaluate foundation model performance.
Objectives:
- Understand approaches to evaluate foundation model performance (for example, human evaluation, benchmark datasets). (AWS Documentation: What are foundation model evaluations?)
- Identify relevant metrics to assess foundation model performance (for example, Recall-Oriented Understudy for Gisting Evaluation [ROUGE], Bilingual Evaluation Understudy [BLEU], BERTScore).
- Determine whether a foundation model effectively meets business objectives (for example, productivity, user engagement, task engineering).
Domain 4: Guidelines for Responsible AI
Task Statement 4.1: Explain the development of AI systems that are responsible.
Objectives:
- Identify features of responsible AI (for example, bias, fairness, inclusivity, robustness, safety, veracity). (AWS Documentation: Responsible AI)
- Understand how to use tools to identify features of responsible AI (for example, Guardrails for Amazon Bedrock). (AWS Documentation: Amazon Bedrock Guardrails)
- Understand responsible practices to select a model (for example, environmental considerations, sustainability). (AWS Documentation: Cloud sustainability)
- Identify legal risks of working with generative AI (for example, intellectual property infringement claims, biased model outputs, loss of customer trust, end user risk, hallucinations).
- Identify characteristics of datasets (for example, inclusivity, diversity, curated data sources, balanced datasets).
- Understand effects of bias and variance (for example, effects on demographic groups, inaccuracy, overfitting, underfitting). (AWS Documentation: Overfitting)
- Describe tools to detect and monitor bias, trustworthiness, and truthfulness (for example, analyzing label quality, human audits, subgroup analysis, Amazon SageMaker Clarify, SageMaker Model Monitor, Amazon Augmented AI [Amazon A2I]). (AWS Documentation: Amazon SageMaker Clarify)
Task Statement 4.2: Recognize the importance of transparent and explainable models.
Objectives:
- Understand the differences between models that are transparent and explainable and models that are not transparent and explainable.
- Understand the tools to identify transparent and explainable models (for example, Amazon SageMaker Model Cards, open source models, data, licensing). (AWS Documentation: Amazon SageMaker Model Cards)
- Identify tradeoffs between model safety and transparency (for example, measure interpretability and performance).
- Understand principles of human-centered design for explainable AI.
Domain 5: Security, Compliance, and Governance for AI Solutions
Task Statement 5.1: Explain methods to secure AI systems.
Objectives:
- Identify AWS services and features to secure AI systems (for example, IAM roles, policies, and permissions; encryption; Amazon Macie; AWS PrivateLink; AWS shared responsibility model). (AWS Documentation: Shared Responsibility Model)
- Understand the concept of source citation and documenting data origins (for example, data lineage, data cataloging, SageMaker Model Cards).
- Describe best practices for secure data engineering (for example, assessing data quality, implementing privacy-enhancing technologies, data access control, data integrity). (AWS Documentation: Security best practices for Amazon S3)
- Understand security and privacy considerations for AI systems (for example, application security, threat detection, vulnerability management,
infrastructure protection, prompt injection, encryption at rest and in transit).
Task Statement 5.2: Recognize governance and compliance regulations for AI systems.
Objectives:
- Identify regulatory compliance standards for AI systems (for example, International Organization for Standardization [ISO], System and Organization Controls [SOC], algorithm accountability laws).
- Identify AWS services and features to assist with governance and regulation compliance (for example, AWS Config, Amazon Inspector, AWS Audit Manager, AWS Artifact, AWS CloudTrail, AWS Trusted Advisor). (AWS Documentation: Security, identity, and compliance)
- Describe data governance strategies (for example, data lifecycles, logging, residency, monitoring, observation, retention). (AWS Documentation: Data Governance)
- Describe processes to follow governance protocols (for example, policies, review cadence, review strategies, governance frameworks such as the Generative AI Security Scoping Matrix, transparency standards, team training requirements). (AWS Documentation: Generative AI Security Scoping Matrix)
4. AWS Whitepapers and Blogs
– Read AWS Whitepapers and Blogs for In-Depth Knowledge
AWS regularly publishes whitepapers and blog posts that explore AI/ML advancements, best practices, and ethical considerations. Reading AWS AI/ML whitepapers can deepen your understanding of topics such as:
- Model interpretability and bias mitigation in AI.
- Security and compliance considerations for AI applications.
- Scalability and cost optimization for machine learning workloads.
Additionally, following the AWS Machine Learning Blog keeps you updated on new features, enhancements, and industry trends—knowledge that could be useful in the exam.
5. Focus on Key AWS AI/ML Services
– Prioritize High-Weightage Services
Certain AWS AI/ML services appear frequently in the exam. Focus on deeply understanding the following:
- Amazon SageMaker – Model training, deployment, and hyperparameter tuning.
- Amazon Rekognition – Image and video analysis capabilities.
- Amazon Comprehend – NLP-based sentiment analysis and entity recognition.
- Amazon Personalize – Building recommendation systems.
- Amazon Forecast – Time-series forecasting.
– Understand How Services Work Together
AWS AI/ML services often integrate with other AWS tools, such as Amazon S3, AWS Lambda, AWS Glue, and IAM. For example:
- Storing training data in S3 before using SageMaker.
- Securing ML models using IAM roles and policies.
- Triggering Lambda functions for serverless ML workflows.
6. Time Management
– Practice Time Management During Practice Exams
AWS exams are time-bound, so learning to pace yourself is crucial. During practice exams:
- Set a timer to match the real exam conditions.
- Avoid spending too much time on difficult questions—mark them and return later.
- Focus on answering high-confidence questions first before tackling complex ones.
– Create a Study Schedule
Consistency is key to retaining information. Develop a structured study plan by:
- Allocating specific days for different topics (e.g., AI services on Monday, Security on Wednesday).
- Breaking down the syllabus into manageable sections.
- Reviewing weak areas more frequently to strengthen understanding.
7. Review Key Concepts Regularly
– Use Flashcards or Summaries
To reinforce learning, create flashcards summarizing key concepts, service capabilities, and best practices. Reviewing flashcards daily helps retain crucial information, making recall easier during the exam.
– Teach the Concepts to Others
One of the most effective ways to solidify understanding is by teaching what you’ve learned. Join a study group, explain concepts to a friend, or even write a short blog post about a topic. Teaching forces you to break down complex ideas, improving conceptual clarity and retention.
Conclusion
The AWS Certified AI Practitioner exam serves as a pivotal stepping stone for both technical and business professionals seeking to validate their foundational knowledge in the rapidly evolving landscape of artificial intelligence and machine learning on the AWS platform. By mastering core ML concepts, gaining practical experience with key AWS AI/ML services like SageMaker, Rekognition, and Comprehend, and understanding the critical aspects of security, compliance, and MLOps, you’ll be well-prepared to not only pass the exam but also apply your expertise to real-world business challenges.
Remember, this certification is more than just a credential; it’s a testament to your commitment to staying at the forefront of AI/ML innovation. Use the resources provided, engage in hands-on practice, and consistently review the essential concepts outlined in this cheat sheet. Embrace the journey of continuous learning, and you’ll find yourself empowered to leverage the transformative power of AWS AI/ML to drive impactful solutions and advance your career. Your dedication and preparation will undoubtedly pave the way for your success in achieving the AWS Certified AI Practitioner certification and beyond.